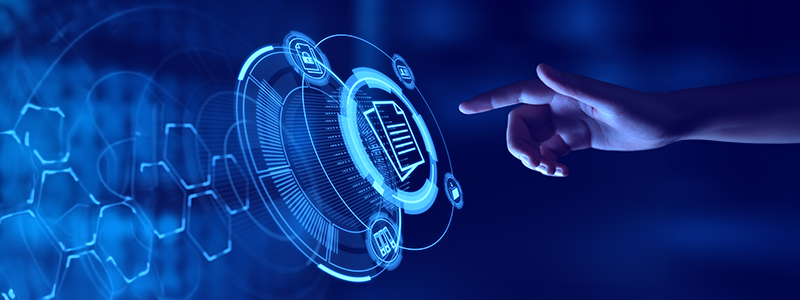
Tackling Layout Variability in Data Extraction Using AI
Data extraction is a critical component of modern data processing pipelines. Businesses across industries rely on valuable information from a range of documents to optimize their processes and make informed decisions.
One commonly employed method for data extraction is the traditional template-based approach. This technique involves creating predefined templates or rules that define the expected structure and data fields within the documents. These templates instruct the extraction system on where and how to locate and extract the relevant data fields. The extraction system matches the document against these templates and extracts the data accordingly.
When using traditional template-based data extraction, various aspects need to be considered to ensure seamless data retrieval from such documents, such as:
- Document structure inconsistencies that can hinder the extraction process.
- The time-intensive nature of template creation, which demands significant resources.
- The potential for errors during the extraction procedure, posing a risk to data accuracy.
- Scalability issues that may limit the ability to efficiently handle a growing volume of documents.
Maximum Accuracy and Efficiency: The Impact of Automated Data Extraction
If we consider that creating a template for a single invoice takes approximately 20-30 minutes and there are 20 invoices with varying layouts, it would require a total of 30 * 20 = 600 minutes, equivalent to 10 hours, to complete the template creation process. This time-consuming process highlights the need for more advanced and efficient data extraction techniques to manage diverse document layouts.
Therefore, modern businesses are exploring a hybrid approach that combines the efficiency of template-based data extraction with the power of advanced language models, such as OpenAI’s GPT or other similar large-scale Language Models (LLMs), to streamline the process of data extraction and tackle the problem of creating templates. Integrating generative AI into the data extraction pipeline can significantly reduce the time and effort required for template creation.
That’s where Astera ReportMiner comes in. AI-powered data extraction in ReportMiner can quickly and accurately extract data from a variety of document types. This feature allows extracting data from purchase orders and invoices with varying layouts without hassle.
Use Case: Automating Purchase Order Data Extraction with Astera ReportMiner
Let’s consider a use case. SwiftFlow Services Inc. (SFS) must manage a daily influx of purchase orders from various vendors received via email. Each day, they receive approximately 10 to 20 purchase orders, with each vendor presenting a unique purchase order layout.
SFS aims to extract specific fields from these purchase orders and store the data in a database for further analysis, such as evaluating vendor performance, identifying cost-saving opportunities, and optimizing supply chain management.
SFS wanted an efficient and streamlined solution that could effortlessly extract the required information without requiring manual template creation. Therefore, they chose Astera’s AI-powered data extraction solution. Users must only specify the document type and desired layout for extraction, and the system harness AI’s context-building ability to extract the information and generate templates consisting of regions and fields using heuristics.
The tool automatically creates templates for all sources within a folder at the project level. Acknowledging the importance of human feedback, the system stores any problematic templates (RMDs) that require user adjustments in a designated folder.
After RMD verification and customization per business requirements, users can create a workflow to loop through these RMDs and write the extracted data to a destination. A Data Quality Rules object further enhances efficiency by ensuring the extracted data adheres to the specified business rules, resulting in faster and more accurate data retrieval.
By simplifying and automating the data extraction process, SFS can reduce manual labor, improve the accuracy of extracted data, and focus on more critical tasks in its data processing pipeline. Check out this video to learn more:
If you want to learn more about ReportMiner, contact our sales team to schedule a demo today.