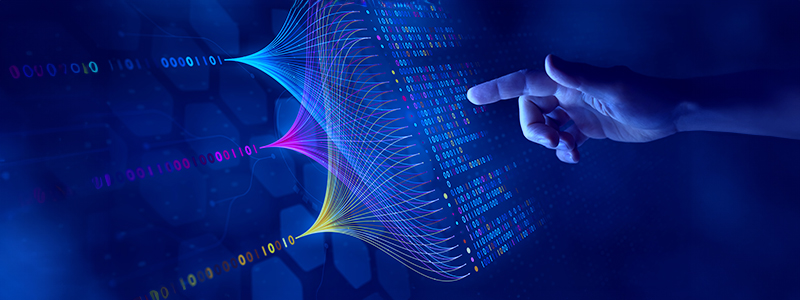
5 Best Practices for Big Data Integration
A staggering amount of data is created every single day – around 2.5 quintillion bytes, according to IBM. In fact, it is estimated that 90% of the data that exists today was generated in the past several years alone. Talk about an explosion!
The world of big data can unravel countless possibilities. From driving targeted marketing campaigns and optimizing production line logistics to helping healthcare professionals predict disease patterns, big data is powering the digital age. However, with monumental volumes of data come significant challenges, making big data integration essential in data management solutions.
What is Big Data Integration?
Big data integration is the process of consolidating and merging data from various sources, which may be structured or unstructured, batch or real-time, and stored on-premises or in the cloud. The objective is to create a single, comprehensive view of the data, enabling organizations to make informed business decisions. Data from different sources can be run through an ETL (extract, transform, and load) engine. Data integration platforms can also simplify the process of loading data into a data pipeline.
Why Does Big Data Integration Matter?
The thing about big data is that it arrives in various shapes and forms, often making it difficult to consolidate, analyze, and put to good use. This is where big data integration comes in, stitching it all together while also ensuring its credibility, timeliness, and accuracy. Without ensuring proper integration, chances are we would be swimming in a sea of disjointed information chunks – not quite the recipe for a data-driven revolution!
Big data integration can provide substantial developments for your business such as:
- Improved Decision-Making: Big data integration provides a unified view of data. It allows businesses to make better decisions based on accurate and up-to-date information.
- Increased Efficiency: By automating the data integration process, businesses can save time and money, and reduce the risk of errors associated with manual data entry.
- Enhanced Customer Experience: Big data integration can help organizations gain a better understanding of their customers. This leads to more personalized and targeted marketing campaigns, improved customer service, and increased customer loyalty.
- Improved Business Insights: By combining data from multiple sources, businesses can gain deeper insights into their operations, enabling them to identify trends, opportunities, and potential risks.
Best Practices for Successful Big Data Integration
Big data integration is a crucial component of any modern business strategy. It provides a unified view of data and enables informed decision-making. By following these five best practices businesses can successfully integrate their data, improve efficiency, and gain valuable insights into their operations.
1. Ensure Data Quality Management
Ensuring that the data being integrated is of high quality is one of the most critical aspects of big data integration. Poor data quality can lead to incorrect conclusions and ultimately, poor business decisions.
Data profiling is one of the key steps data quality management. This process involves analyzing the data to identify inconsistencies and errors. By identifying missing or incomplete data in customer data from multiple sources, organizations can ensure that their data is accurate and complete.
Data cleansing is another important practice for data integration. This process involves correcting or removing any errors identified during the data profiling process. For instance, data cleansing can help correct any spelling errors or duplicates in a data set.
Data validation is essential to ensure the data is accurate and meets predefined rules. For example, data validation can help ensure that the data is compliant with regulatory requirements. Organizations should ensure high-quality data to make informed business decisions based on accurate insights.
2. Enhance Security Measures
Security is a crucial consideration when integrating big data. Data may contain sensitive information that requires protection from unauthorized access. There are several measures that organizations can take to ensure data security during integration.
Encryption is an approach that involves converting the data into an unreadable format that requires a decryption key to be read. For instance, when integrating employee data from various sources, one can use encryption to protect sensitive information such as social security numbers.
Access controls are another crucial aspect of data security during integration. These controls determine who can access the data by setting permissions. When integrating customer data from multiple sources, access controls can limit access to authorized personnel, reducing the risk of data breaches.
Secure data transfer protocols are also crucial measures in ensuring data security during integration. These protocols involve using secure protocols such as HTTPS, FTPS, and SFTP to transfer the data. Secure data transfer protocols can help ensure that the data is transmitted securely and protected from unauthorized access, for example.
Implementing these measures can help organizations protect their data during integration, maintain the confidentiality and integrity of sensitive information, and reduce the risk of data breaches.
3. Build Scalable Solutions
A critical factor in big data integration is scalability, as the amount of data being integrated can increase rapidly. Organizations must ensure that the integration process can handle the increased workload to ensure seamless integration.
Cloud-based integration solutions provide a scalable option by offering on-demand computing resources. For instance, a cloud-based integration solution can help handle an increased workload by providing additional computing resources as needed. This approach enables organizations to integrate more data without having to invest in expensive hardware infrastructure.
Distributed computing is another way to achieve scalability in big data integration. This process involves dividing the workload among multiple nodes to increase performance. Large volumes of data can be processed in parallel with distributed computing, reducing the overall processing time. This approach is particularly useful when integrating data from multiple sources and the amount of data being integrated is substantial
By following these measures, organizations can ensure that their integration process can handle large volumes of data seamlessly, reducing processing time and ensuring scalability. This approach also enables organizations to integrate data without incurring significant infrastructure costs.
4. Conduct Thorough Testing
Testing is a critical aspect of big data integration that ensures the accuracy of the integrated data and the effectiveness of the integration process. To ensure successful testing, organizations can follow these measures:
Automated testing can help organizations save time and resources in the testing process by using tools to automate the testing process. For ensuring that the data is accurately integrated, automated testing is particularly useful. For instance, automated testing can help identify any errors that may occur during the integration process.
Data sampling is another way to ensure successful testing in big data integration. This process involves selecting a subset of data for testing instead of testing the entire dataset. Organizations can identify any data quality issues before the data is fully integrated by testing a subset of data. This can help reduce testing time and increase efficiency, enabling organizations to identify and resolve issues quickly.
Performance testing involves testing the integration process’s performance to ensure that it can handle the expected workload. For example, organizations can use performance testing when integrating log data from multiple sources to ensure the integration process can handle the large volume of data. Performance testing is essential to ensure that the integration process can handle the expected workload and identify any issues that may arise.
By ensuring thorough testing measures, organizations can ensure that their integration process is accurate and effective. Testing is critical in big data integration to ensure data quality and minimize the risk of making incorrect business decisions based on inaccurate data.
5. Implement Effective Data Governance
Data governance is critical to managing the availability, usability, integrity, and security of data. Organizations can ensure effective data governance by implementing the following measures.
Data cataloging can help manage the data by creating a metadata repository that provides information about the data being integrated. Data cataloging can help ensure data is well-managed and easily accessible. For example, when integrating customer data from multiple sources, data cataloging can ensure that all customer data is available in one central location.
Data lineage involves tracking the movement of data from its source to its destination. Data lineage ensures the accuracy of data and its traceability back to its original source. This practice can check if data is compliant with regulatory requirements.
Data stewardship involves assigning responsibility for managing data to specific individuals or teams. It can ensure well-managed data and prompt addressing of any issues. For instance, if an organization is integrating HR data from multiple sources, it addresses any issues with the data quickly
Breaking it down, the crux of solving the big data puzzle lies in effective data integration. Big data integration is a seamless way to weave together crucial insights from an unimaginable amount of data. It is a complex process that requires careful planning and execution. Organizations can ensure the data being integrated is of high quality, secure, scalable, well-tested, and well-governed by following these best practices. Integration is the bridge between diverse data and valuable insights, and by mastering this art, we’re well on our way to creating a data-driven future!