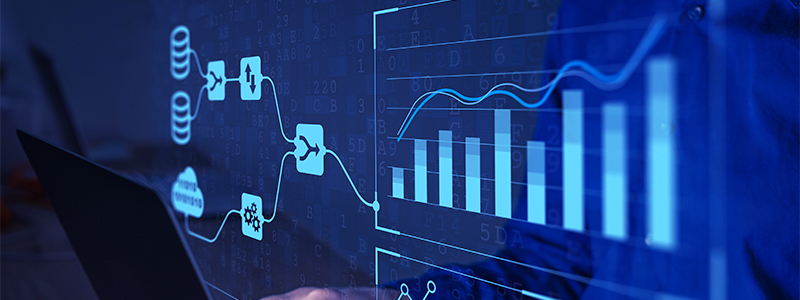
The Rise of Agentic Automation: What It Means for Enterprises
By 2025, one in four enterprises using Gen AI will have AI agents in place, and that number will double by 2027. As organizations race to integrate these intelligent technologies, the spotlight is on agentic automation, a transformative approach reshaping how businesses operate.
Right now, enterprises are at a key turning point. Some are already deploying AI agents to streamline operations, improve customer interactions, and drive innovation, while others are still exploring how to integrate this technology effectively. The path forward is clear—embedding agentic automation into core business functions will empower organizations to adapt, innovate, and stay ahead of the curve.
What Is Agentic Automation?
Agentic automation refers to the use of autonomous AI agents that can independently analyze, plan, and execute tasks without human intervention. Unlike traditional robotic process automation (RPA), which relies on predefined workflows and rule-based logic, agentic process automation enables software agents to learn, adapt, and make decisions dynamically.
At its core, agentic process automation (APA) combines:
- Artificial Intelligence (AI): Powers autonomous agents with reasoning, learning, and contextual understanding.
- Machine Learning (ML): Continuously enhances agent performance through data-driven insights and pattern recognition.
- Natural Language Processing (NLP): Enables AI agents to understand, interpret, and respond to human language with accuracy.
- Multi-Agent Systems: Coordinates multiple AI agents to collaborate, adapt, and execute complex workflows autonomously.
- Adaptive Workflows: Dynamically adjusts processes based on real-time data, improving efficiency and responsiveness.
With these capabilities, agentic process automation represents a significant evolution in automation, moving beyond task-based execution to holistic, intelligent decision-making.
Benefits of Agentic Process Automation
Key benefits of agentic automation include:
- End-to-End Task Automation: AI agents handle entire workflows independently, analyzing data, making decisions, and executing tasks without delays or manual intervention.
- Dynamic Process Adaptation: Workflows adjust in real-time based on new inputs, exceptions, and evolving conditions. AI agents continuously refine their decision-making to optimize performance.
- Instant Responsiveness: Tasks are executed the moment data becomes available, ensuring quick turnarounds and allowing businesses to react faster to changes and opportunities.
- Smarter Resource Allocation: Workloads are intelligently distributed based on urgency and capacity, maximizing efficiency while preventing bottlenecks and unnecessary operational costs.
- Proactive Issue Resolution: Anomalies and inefficiencies are detected and addressed before they escalate. AI agents continuously monitor operations, applying corrective actions when needed.
- Built-In Security and Compliance: Sensitive data is managed securely, with automated enforcement of compliance policies, access controls, and audit trails to reduce risk and ensure regulatory adherence.
- Personalized Experiences: AI agents tailor responses, automate requests, and provide personalized recommendations based on user behavior and preferences, improving engagement and satisfaction.
- Uninterrupted Operations: Automation minimizes reliance on specific individuals, ensuring business continuity even during workforce changes, system updates, or unexpected disruptions.
How Does Agentic Automation Differ from Traditional Automation?
For years, businesses have relied on automation to improve efficiency and reduce costs. It started with Robotic Process Automation (RPA), which used bots to mimic human interactions with systems—logging into applications, copying data, and processing transactions. While effective for rule-based, repetitive tasks, RPA was rigid. Bots would break when applications changed, and any deviation from predefined rules required human intervention, limiting its adaptability.
To overcome these challenges, organizations adopted Intelligent Automation (IA), which combined RPA with AI, ML, NLP, and computer vision. IA could process unstructured data, make predictive decisions, and continuously improve over time. However, despite its intelligence, IA still required human oversight—AI could analyze and recommend actions, but humans remained essential for validation.
Now, agentic process automation marks a shift from automation as an assistant to automation as an autonomous agent. Unlike RPA, which follows predefined rules, and IA, which enhances decision-making, agentic automation enables AI-driven agents to sense, decide, and act independently. These agents adapt dynamically, collaborate with other AI systems, and optimize processes in real time, requiring minimal human intervention. With this technology, businesses move beyond automation that simply executes tasks to AI-driven operations that self-manage and continuously evolve.
Feature | RPA | Intelligent Automation (IA) | Agentic Process Automation (APA) |
Function | Automates rule-based tasks | Enhances automation with AI | Fully autonomous decision-making |
Learning Capability | None | Limited (Machine Learning) | Continuous self-learning & adaptation |
Decision-Making | None | AI-assisted (suggestions) | Fully independent |
Flexibility | Low | Medium | High |
Human Involvement | High | Moderate | Minimal |
Scalability | Limited | Expands with AI | Fully scalable & adaptive |
How does Agentic Automation Work?
Step 1: Understanding the Environment
Agentic process automation starts with AI agents gathering data from various sources, including databases, APIs, documents, and live system interactions. Using AI, ML and NLP, they extract insights, recognize patterns, and interpret context, just as a human would. This allows them to work with both structured and unstructured data, adapting to different formats and business conditions.
Step 2: Selecting the Best Action
With a clear understanding of the data, agents determine the most effective course of action. They use AI-driven reasoning, reinforcement learning, and predictive analytics to assess different possibilities and choose the optimal path. This means that rather than executing the same action every time, the agents assess the context, weigh possible outcomes, and choose the most efficient path.
Step 3: Executing & Orchestrating Tasks
Once a decision is made, the agents execute tasks by interacting directly with enterprise applications, processing transactions, or triggering system actions. If unexpected changes occur, AI agents in APA dynamically adjust their approach, finding alternative methods to complete a task when faced with unexpected conditions.
Step 4: Coordinating Workflows Smoothly
APA enables multiple agents to work together, communicating and sharing data to optimize workflows. These agents organize tasks, distribute work efficiently, and ensure smooth execution of complex processes without delays. Their ability to coordinate allows automation to extend across departments, systems, and even external partners
Step 5: Adapting and Improving
APA is not static—it continuously improves. Through reinforcement learning and feedback loops, AI agents refine their models, enhance decision-making accuracy, and optimize workflows. This self-learning capability ensures that processes adapt to new business needs, regulatory changes, and system updates without requiring manual reconfiguration.
Challenges and Solutions in Agentic Process Automation
As businesses move toward agentic process automation, they encounter challenges in implementation, governance, and integration. Addressing these issues requires a structured approach to ensure efficiency, compliance, and long-term success.
Managing Complexity in Multi-Agent Systems
With multiple AI agents working together, ensuring smooth coordination and preventing inefficiencies can be difficult. Without structured interaction protocols, agents may overlap in functions, create bottlenecks, or operate in conflicting ways. Establishing clear governance frameworks, defining roles, and using orchestration tools help streamline collaboration and maintain efficiency in automated workflows.
Ensuring Trust and Transparency
Since agentic process automation operates with minimal human intervention, organizations need visibility into how AI agents make decisions. A lack of transparency can raise concerns about accountability and compliance. Implementing explainable AI (XAI) models allows businesses to understand decision-making processes while logging and monitoring mechanisms provide audit trails and ensure compliance with regulations.
Handling Unpredictable Scenarios
AI agents must be able to respond to unexpected events, such as system failures, data inconsistencies, or regulatory changes. Without adaptability, they may struggle to function effectively in dynamic environments. Developing AI models with advanced reasoning capabilities, incorporating feedback loops, and maintaining human oversight at key checkpoints can ensure smooth adaptation to new conditions.
Integrating with Legacy Systems
Many enterprises rely on outdated infrastructure that may not be compatible with AI-driven automation, leading to deployment challenges. Without proper integration, AI agents may struggle to access critical data or operate across different platforms. API-driven connectors and middleware solutions help bridge this gap, enabling seamless interaction between automated workflows and legacy systems. A gradual, hybrid approach allows businesses to modernize without disrupting ongoing operations, ensuring a smooth transition to agentic process automation.
Ensuring Data Security and Compliance
With AI agents handling large volumes of sensitive data, maintaining security and regulatory compliance becomes even more critical. A lack of robust security measures can expose organizations to data breaches and legal risks. Implementing encryption, role-based access controls, and real-time compliance monitoring can safeguard data integrity. AI-driven security frameworks can proactively detect and mitigate potential threats.
Scaling Automation Efficiently
As organizations continue to incorporate agentic automation across business units, managing growing workloads while maintaining performance consistency can be challenging. Without scalable infrastructure, automation may become inefficient or resource-intensive. Deploying cloud-based APA solutions provides adaptive computing power, ensuring that automation scales efficiently while maintaining high performance. Dynamic resource allocation allows systems to adjust to demand fluctuations without compromising speed or reliability.
How Businesses Can Prepare for APA Adoption
The transition to agentic process automation is more than a technological upgrade—it requires strategic planning, cultural shifts, and infrastructure readiness. To successfully incorporate agentic automation, businesses must focus on key areas that ensure smooth implementation and long-term success.
- Assess Automation Readiness
Before integrating agentic process automation, organizations must evaluate their current automation landscape. Identifying repetitive, data-driven processes that require minimal human intervention can help pinpoint where agentic automation can drive the most impact. Businesses should also assess existing data quality, governance frameworks, and technological infrastructure to ensure compatibility with key solutions. - Build a Strong AI Strategy
A clear AI strategy helps define the scope of agentic automation, the level of autonomy required, and risk mitigation measures. Companies should establish guidelines for AI governance, ethical considerations, and regulatory compliance to prevent unintended consequences. - Invest in Scalable APA Infrastructure
A robust agentic automation platform should seamlessly integrate with enterprise systems, handle large-scale process automation, and allow for adaptability. Cloud-native platforms with low-code capabilities empower business users to automate workflows without deep technical expertise. Choosing a solution with pre-built connectors and APIs ensures smooth integration with existing enterprise applications. - Enable Workforce Upskilling
With agentic automation taking over decision-making and process execution, human roles will evolve. Organizations should invest in reskilling and upskilling programs to help employees transition to higher-value tasks such as managing AI models, interpreting insights, and strategic planning. Encouraging a culture of AI literacy ensures that employees can collaborate effectively with intelligent agents. - Implement Change Management Strategies
Automation adoption often meets resistance due to fears of job displacement or loss of control. Transparent communication about agentic automation’s role in enhancing efficiency rather than replacing employees is critical. Organizations should involve stakeholders early, highlight the benefits of agentic process automation, and create structured change management initiatives to ensure a smooth transition. - Define Metrics for Success
Measuring agentic process automation’s impact is essential for continuous improvement. Businesses should track efficiency gains, cost reductions, error reduction, and process optimization to assess the effectiveness of their automation initiatives. Establishing key performance indicators (KPIs) helps refine automation strategies and maximize ROI.
Embracing the Future with Agentic Automation
Agentic process automation is redefining how businesses operate, creating a digital workforce that is intelligent, autonomous, and adaptive. As AI-driven agents take on complex tasks, organizations can scale faster, streamline operations, and unlock new efficiencies. The shift toward agentic process automation is not just about automation—it’s about transforming business processes to be more agile and future-ready.
Astera empowers enterprises to leverage the full potential of agentic automation with a powerful, scalable, and user-friendly platform. Businesses can rapidly develop AI agents in hours, seamlessly connect to enterprise data sources, and integrate with any leading LLM, from OpenAI to in-house models. With Astera’s renowned ETL technology, AI agents connect directly to enterprise data without complex integrations, enabling them to work on real business data effortlessly. Its visual, drag-and-drop environment ensures that every team, from HR to sales, can create and optimize AI-driven solutions without technical barriers.
Want to see agentic automation in action? Visit our product page to discover how it’s transforming the way teams work.