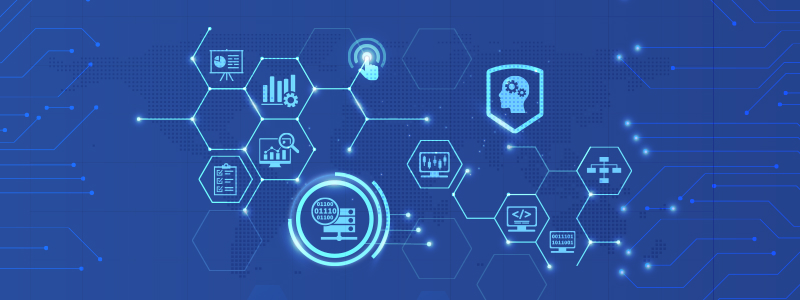
What are Agentic Workflows?
Organizations are moving beyond simple automation towards a future where systems are intelligent enough to tackle complex tasks with minimal human intervention. Agentic workflows are the driving force behind this shift.
According to Gartner, a staggering 33% of enterprise software applications are projected to integrate agentic AI by 2028, enabling them to autonomously make decisions for as much as 15% of routine work. The projection highlights a significant shift toward agentic workflows becoming embedded in the very fabric of enterprise software, paving the way for a deeper and more direct interaction with AI. As Satya Nadella, CEO of Microsoft, aptly puts it,
“AI agents will become the primary way we interact with computers in the future. They will be able to understand our needs and preferences and proactively help us with tasks and decision-making.”
But what exactly is an agentic workflow? And why is it gaining prominence? Let’s find out.
What are agentic workflows?
An agentic workflow is a series of actions orchestrated by autonomous agents working together to achieve specified goals.
An agent within an agentic workflow is an entity that:
- “Sees” and “understands” its environment (interacts with it through data or context)
- Goal-oriented, i.e., designed to achieve specific predefined tasks
- Operates autonomously and doesn’t require constant human intervention for decision-making
- Has the ability to learn from experiences and improve over time
Typically, these are AI agents, software tools, human experts, or even simple scripts. Agentic workflows comprising solely of AI agents are also referred to as agentic AI workflows or AI agentic workflows and are used interchangeably.
The evolution of agentic workflows can be traced back to the pioneering research in multi-agent systems (MAS) and distributed artificial intelligence (DAI), exploring how multiple intelligent agents could collaborate to solve complex problems and laying the groundwork for what would eventually become agentic workflows. Today, these workflows integrate the lessons learned from multi-agent systems with modern AI capabilities, allowing for dynamic, context-aware decision-making.
Traditional workflows vs. AI agentic workflows
Organizations face increasingly complex and dynamic challenges, reducing the overall utility of traditional, rule-based workflows. Building on this limitation, agentic workflows offer a significant advantage as they are built to automatically adjust how tasks are handled.
Traditional workflows typically follow a fixed approach where every step is predetermined and relies on human intervention for exceptions or changes. These systems work well in controlled environments but struggle when faced with unexpected variations or a surge in data.
In contrast, agentic workflows leverage autonomous AI agents that can adapt in real time. Rather than sticking to rigid rules, these workflows continuously learn from their environment, dynamically adjust processes, and re-prioritize tasks based on current conditions.
How do agentic workflows work?
In an agentic workflow, agents communicate, share information, and perform specific tasks based on their capabilities, all orchestrated by the workflow to achieve an outcome that might otherwise be too intricate for a single agent to handle effectively.

How an agentic workflow works
The orchestration of tasks involves a central system or framework that defines:
- the sequence of tasks
- the dependencies between agents
- the rules for information exchange
The workflow ensures that the output of one agent seamlessly feeds into the next, creating a cohesive and efficient process. Here’s how AI agentic workflows normally work:
Specialized roles
Each agent is designed to perform a specific function and brings its unique skills and knowledge to the process, focusing on its own task. The roles must be clearly defined so that each task is handled by the most capable component of the system.
Inter-agent communication
Communication enables agents to share real-time insights and intermediate results, ensuring that the overall workflow remains coherent and adaptive. Agents exchange information using standardized protocols (e.g. HTTP/HTTPS) or APIs.
Task execution
A central orchestrator or coordination mechanism manages the sequence and interdependencies of tasks assigned to the agents. While each agent has a predefined role, the orchestrator controls the execution schedule by determining the optimal order and timing for these tasks. It monitors progress and adjusts the workflow as conditions change, aligning all tasks to work in harmony toward the common objective.
Depending on the complexity of the task, agents may operate in parallel or in a sequential manner.
Feedback and optimization
A key aspect of this workflow is its continuous collection of performance metrics and outcome data. Individual agents then use this feedback to adjust their operations and quickly adapt to new information in near real-time.
Key components of agentic workflows
To understand what makes up an agentic workflow, it’s important to realize that an agentic workflow is a dynamic, multi-layered ecosystem where autonomous AI agents collaborate with tools, data, and even human overseers to drive complex tasks toward a defined goal.

Components of an agentic workflow
The agentic workflow architecture comprises of:
AI agents
This autonomous entity, designed to perceive its environment, reason, plan, and act to achieve specific goals, is the core of agentic workflows. AI agents can be single-purpose (e.g., a chatbot answering queries) or multi-functional, capable of reasoning, learning, and adapting based on inputs and feedback.
AI agents utilize chain-of-thought and multi-step planning techniques to break down high-level objectives into smaller, actionable tasks. Techniques such as ReAct, Self-Refine, and even emerging frameworks like AutoCoA (which internalizes chain-of-action generation) help agents decide what to do next.
Large language models (LLMs)
Large language models (LLMs), such as GPT-4, are the core intelligence behind AI agents. LLMs are tasked with processing natural language inputs, generating responses, and decision-making. They enable agents to understand context and retrieve information to perform reasoning tasks, making them essential for agentic workflows.
Perception systems
For any agent to function autonomously, it must have a robust mechanism for understanding its environment. Perception systems typically comprise of:
- Data ingestion and sensory inputs: AI agents pull data from diverse sources, such as APIs, databases, sensor networks, or real-time web feeds, to get an up-to-date picture of their operational context.
- Memory architecture: Includes short-term memory (to maintain the context of a current session) and long-term memory (to learn from historical interactions) so that agents can make context-aware decisions and continuously improve.
- Natural language processing (NLP): AI agents use NLP to interpret unstructured text, ask clarifying questions, and understand complex instructions.
Prompt engineering and UI design
The human–machine interface defines how effectively agents can be instructed and how transparently they operate. They allow human operators to understand the agent’s thought process, intervene when necessary, and trust the system’s autonomous decisions.
Crafting precise, context-rich AI prompts is an art in itself. The better the prompt, the more reliably the agent can perform its reasoning and planning functions. So, effective prompt engineering leads to useful and relevant responses from LLMs.
Planning and task decomposition
For complex tasks, AI agents must be able to break down objectives into manageable sub-tasks. This is what agentic workflows are excellent at and why they’re gaining so much traction.
Planning involves strategizing the best course of action, while task decomposition is responsible for organizing multi-step workflows. This process is guided by advanced prompt engineering that instructs the AI to think step-by-step.
Tool and external integration
To complete certain tasks, AI agents may need to extend their capabilities beyond their built-in knowledge. This requires access to external tools and software systems, such as web search engines or specific APIs.
Multi-agent communication
In more advanced systems where multiple agents work towards a common goal, seamless communication is key. AI agents rely on standardized interfaces to collaborate, share information, and divide work. This leads to distributed problem solving where each agent handles a distinct part of a problem. For example, one gathering data, another planning the sequence of actions, and yet another executing the tasks.
Internal and human-guided feedback
Agentic workflows are inherently adaptive, which means they improve over time. However, for this to happen, mechanisms like internal feedback and human-in-the-loop (HITL) must be implemented. Internal feedback involves self-assessment, where an agent refines its outputs based on predefined criteria. Whereas human-guided feedback involves users correcting or steering the agent’s responses so that they align with user intent.
Why agentic workflows outperform individual AI agents
The real question to ask here is, are businesses really moving away from AI agents to AI agentic workflows? Even if organizations are increasingly turning toward agentic AI workflows, it’s not necessarily a complete “shift away” from AI agents, but rather a recognition that for many complex problems, a single AI agent might not be the most efficient or effective solution.
Instead of trying to build one super-smart AI agent that can do everything, agentic workflows are based on building a team of specialized agents that can work together effectively. The upside of this approach is more efficient, reliable, and adaptable solutions for complex real-world problems.
For instance, consider a smart city traffic management scenario. Specialized agents can be deployed for different tasks:
- One agent continuously collects and analyzes data from traffic cameras and sensors to identify congestion or accidents in real-time
- Another agent dynamically adjusts traffic signal timings based on current conditions
- A third agent coordinates with public transportation and emergency services to reroute traffic during critical events
- Additionally, a forecasting agent uses historical and live data to predict future congestion patterns, enabling proactive measures

Agentic workflow example
Clearly, this is a multi-faceted workflow, and trusting a single AI agent to achieve all these tasks seamlessly would be an overkill. Secondly, individual agents tend to be less robust and adaptable, potentially making them a single point of failure if they encounter an unexpected situation or malfunction. In contrast, agentic workflows are better at managing failures or unforeseen inputs with their collection of specialized agents. Even if one agent experiences an issue, another agent steps in and keeps the system operational.
Agentic workflows use cases
Agentic workflows leverage modular design and dynamic tool integration to unlock versatile AI capabilities across multiple domains. These systems empower agents to adjust their decision-making autonomy in response to evolving challenges. Integrated human feedback further refines their performance, ensuring that each configuration is fine-tuned for optimal effectiveness across a wide range of industries. Here are some use cases catered by agentic AI workflows:
Agentic RAG
AI agents in agentic workflows leverage the retrieval-augmented generation (RAG) technique to:
- enhance their ability to understand and respond to complex queries
- generate more accurate and informative outputs
- perform tasks that require up-to-date or external knowledge
Agents in this scenario dynamically decide when and how to retrieve information from external knowledge sources based on the task at hand.
Agentic RAG offers several tangible benefits for businesses. It improves efficiency by automating complex information-seeking and processing tasks that previously required substantial human effort. This leads to reduced operational costs and faster turnaround times for various processes, such as customer support inquiries or research projects.
Customer service and support automation
Agentic workflows are used in customer services and support functions to improve response times and free up human agents for high-value tasks. They are designed to interact with customers through natural language, providing immediate responses 24/7. Since AI agents are capable of handling a large volume of customer inquiries, they can:
- provide instant responses to common questions
- guide users through troubleshooting steps
- escalate complex issues to human agents
Agentic coding/software assistants
In the case of software development and coding, AI agents are designed to understand natural language instructions and code, enabling them to assist developers with a wide range of tasks throughout the development lifecycle. The overall impact is a more efficient, productive, and higher-quality software development experience. AI software assistants can:
- identify bugs and offer suggestions
- generate code snippets based on context
- automate repetitive coding tasks
Operational process automation
Operational processes such as document processing and management, invoice processing, HR onboarding, and supply chain coordination involve numerous repetitive and multi-step tasks that can be automated to a very high degree using agentic workflows. Utilizing AI agents that understand complex procedures, businesses can:
- automate data extraction from various documents
- maintain optimal inventory levels by automatically monitoring stock levels, forecasting demand, and reordering
- automate HR an administrative work, e.g. processing new hire documentation or updating employee records
How AI agent builders help with agentic workflows
Agentic workflows are autonomous, multi-step processes where AI agents independently plan, decide, and execute tasks. AI agent builders are key enablers of these workflows as they provide the necessary tools and capabilities needed to build smart agents.
In other words, an AI agent builder is essentially a platform that empowers enterprises to build and deploy agentic workflows by simplifying the process of creating and managing AI agents capable of working independently and adapting to new data. For businesses, this opens an array of possibilities for automation, innovation, and improved efficiency across business functions.
Here’s why organizations are turning away from traditional AI development toward a more rapid development approach enabled by AI agent builders:
- Low-code development via intuitive interfaces and pre-built modules allow non-technical experts to design and deploy AI agents quickly
- Seamless integration with enterprise systems enables agents to easily access and work with data
- Rapid prototyping and iteration mean teams can speed up testing
- Businesses can finally empower subject matter experts to build custom agents that truly reflect and address specific business needs
Astera—the fastest way to build, test, and run AI agents
Astera empowers enterprises to harness the power of AI by transforming their data into actionable intelligence. With our visual drag-and-drop designer, your teams can rapidly build, test, and deploy intelligent AI agents that are tailored to your unique business needs.
Here’s why enterprises choose Astera:
- Rapid AI agent development that turns ideas into working AI agents in hours
- Seamless integration with enterprise data sources whether databases, files, or APIs
- Scalable and secure platform that supports any LLM—OpenAI, Anthropic, Mistral, Llama, and even your internal models
- AI for everyone enables every team, from HR to customer support to sales and marketing, to create AI solutions without relying on specialized coding teams
- Continuous improvement via a simple drag-and-drop environment that enables anyone to easily iterate, test, and optimize their AI agents
Learn more about how Astera AI Agent Builder can help you build autonomous AI agents in a matter of hours.