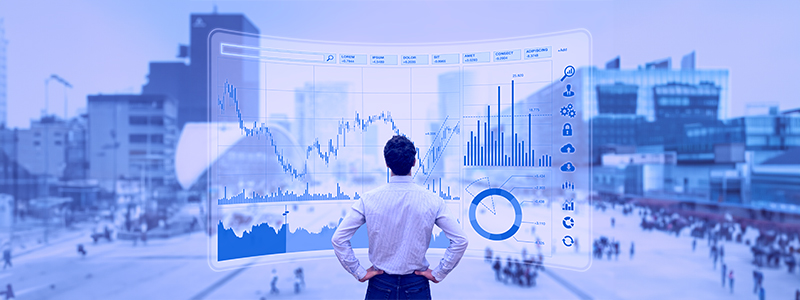
AI and Real-World Evidence (RWE): Extracting Insights from Real-World Health Data
By automating tedious data tasks, AI enables scientists to focus on innovation and discovery
Real-world evidence (RWE) holds significant potential for practitioners to gain insights into the safety and effectiveness of medical products within real-life environments. This valuable resource is derived from real-world data (RWD), encompassing diverse sources like electronic health records (EHRs), claims data, patient-generated data, as well as information from mobile health apps and wearable devices.
Artificial Intelligence and RWE
The transformative effect of Artificial Intelligence (AI) on RWE in healthcare is undeniable. AI enables practitioners to extract meaningful insights from RWE by analyzing large and complex datasets. By leveraging AI, researchers and healthcare providers can identify patterns and trends in real-world data that can inform clinical decision-making, optimize drug development, and improve patient outcomes.
For example, AI is used to analyze EHRs to identify patients at risk for certain conditions or predict treatment responses based on patient characteristics. Additionally, AI is used to analyze social media data and patient-generated data from mobile health apps and wearables to gain a more comprehensive understanding of patient behavior and treatment outcomes.
How AI and RWE Are Changing the Landscape of Medicine
While drug safety regulation remains the most well-known application of real-world data, AI-powered analytics has garnered interest from various stakeholders in the healthcare ecosystem. Biopharmaceutical companies, payors, providers, policymakers, and patients alike are increasingly drawn toward AI-powered RWD’s potential to unlock transformative insights into healthcare outcomes and treatment efficacy.
- Biopharmaceutical companies: The integration of AI-driven RWD analytics is attracting the attention of biopharmaceutical companies. By analyzing real-world data with AI, these companies can optimize drug development and improve patient outcomes.
- Payors: The incorporation of AI in RWD analysis is of great interest to payors, as it enables them to make more informed decisions regarding reimbursement and coverage. The use of AI in RWD analysis can help payors better understand the value of different treatments and interventions.
- Providers: AI-powered RWE analytics can provide providers with insights into patient outcomes and guide clinical decision-making. By analyzing real-world data with AI, providers can identify areas for improvement in patient care and optimize treatment plans.
- Policymakers: The utilization of AI in RWD analysis is also of interest to policymakers, as it can inform health policy decisions and resource allocation. By using AI in RWD analysis, policymakers can better understand the impact of different interventions and make informed decisions about healthcare spending.
- Patients: With the help of AI-powered RWE analysis, patients can make more informed decisions regarding their healthcare. By analyzing real-world data with AI, patients can gain insights into how treatments perform in real-world settings and make more informed choices about their treatment options.
McKinsey projects a top-20 pharmaceutical company can generate $300M annually by integrating advanced RWE analytics over the next three to five years.
Navigating the Complexities of AI-Driven RWE: Key Challenges
The combination of AI and RWE has the potential to transform healthcare by providing more personalized and effective care to patients. However, there are still challenges to be addressed to ensure that these technologies are used ethically, effectively, and responsibly.
- Data quality and standardization: Real-world data can come from various sources, including electronic health records, claims data, and patient-generated data, which may have different formats, coding systems, and levels of completeness. By ensuring data quality and consistency, companies can obtain reliable and meaningful insights.
- Data privacy and security: RWE often contains sensitive patient information, such as their health status, demographics, and behaviors. It is crucial to protect the privacy and confidentiality of this data, especially when using AI algorithms that can potentially identify individuals or infer sensitive information. Using a data extraction solution to secure this data can protect against breaches of privacy.
- Regulatory and legal frameworks: The use of RWE and AI in healthcare is subject to various regulatory and legal requirements, such as data protection laws, ethical guidelines, and validation standards. Solutions that comply with these frameworks allow companies to focus on making faster, more insightful decisions, while AI based extraction solutions do the heavy lifting.
- Interoperability and integration: AI applications that rely on RWE may need to integrate with existing healthcare systems, such as electronic health records, clinical decision support tools, and telemedicine platforms. Finding a solution that can integrate these heterogeneous systems is the key to interoperability.
- Clinical validation and adoption: The utility of AI and RWE in healthcare requires rigorous validation and testing to ensure their safety, efficacy, and clinical utility. A solution that is intuitive and requires a short learning curve allows healthcare providers to put their time where it is most needed: their patients.
End-to-End Data Management: The Ultimate Solution to AI-Driven RWE Challenges
End-to-end data management with automated data extraction can be a game-changer in addressing the challenges posed by AI-powered RWD and RWE in healthcare.
By leveraging advanced technologies such as machine learning and optical character recognition, automated data extraction can streamline the collection and standardization of real-world data from various sources, such as electronic health records, clinical trials, and social media. This can help ensure the quality and consistency of data, reducing the risk of errors and biases that can compromise the accuracy and reliability of insights derived from RWE.
Automating the data extraction process also enhances data privacy and security by minimizing the need for human intervention in handling sensitive information and ensuring compliance with data protection laws and ethical guidelines.
In addition, it can enable interoperability and integration of AI applications with existing healthcare systems, such as EHRs and clinical decision support tools. This can facilitate seamless data exchange and communication between different systems, improving the efficiency and effectiveness of patient care. The automation also accelerates clinical validation and adoption of AI and RWE by providing real-time access to relevant and reliable data for healthcare providers and patients.
By enabling data-driven decision-making and personalized interventions, end-to-end data management with automated data extraction can unlock the full potential of AI-powered RWD and RWE, transforming how modern organizations and practices approach healthcare.
Learn more here to see how Astera can help.