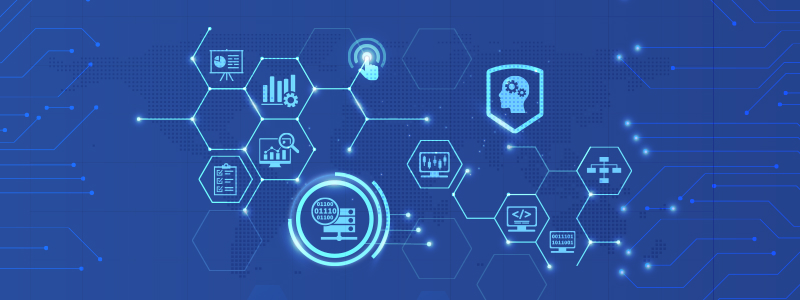
Extracting Actionable Insights from Learning Analytics Data with AI: Enhancing Teaching and Learning
Enhancing Student Success with AI-Driven Learning Analytics
Every school strives for a classroom where every student is engaged, actively participating, and making progress at their own pace. Sounds like a dream, right? Well, it’s not too far off with the help of learning analytics and AI. These tools enable educators to gain insights into student behavior and performance, personalize teaching to student needs, and identify areas where students may struggle.
By leveraging data to inform teaching practices, educators can create a more effective and equitable educational experience for all students. Moreover, with learning analytics, teachers can harness data to empower students and enable them to thrive in an ever-changing world.
The Power of Learning Analytics
In today’s digital education age, learning analytics is a powerful tool for improving student success.
According to a report by MarketsandMarkets, the global learning analytics market is expected to reach US$8.2 billion at a compound annual growth rate (CAGR) of 21.5% during 2019-2024. This growth is being fueled by the adoption of digital learning tools post-pandemic. These tools offer remote access to educational materials, foster seamless communication between teachers and students, and enable individualized instruction.
With these advanced technologies, educational institutes can analyze a wealth of data to gain insights into student conduct and progress. Through learning analytics, instructors can tailor their teaching methods to better support students’ individual needs. They can also identify at-risk students early on to provide personalized interventions for academic success. The potential of learning analytics is enormous and expected to proliferate in the coming years.
Using Learning Analytics to Customize Teaching to Student Needs
As the traditional one-size-fits-all approach to teaching becomes outdated, educators are now turning to technology to improve student outcomes. Consequently, learning analytics has emerged as a widely accepted tool that empowers educators to tailor their teaching strategies and provide targeted support, unlocking the potential of every student.
Here are some ways that learning analytics helps to customize the learning experience:
Identifying Student Learning Styles
Learning analytics can provide insights into how individual students learn best. Analyzing data on student interactions with course materials and assessments can help teachers identify patterns in student learning styles. They can then adjust their teaching methods accordingly. For example, if a student learns best through visual aids, educators can incorporate more visual elements into their teaching materials.
Providing Targeted Support
Educators can use learning analytics to identify at-risk students needing additional support to succeed. By analyzing student performance, behavior, and engagement data, educators can identify struggling students and provide targeted interventions to help them succeed.
For example, suppose students are not engaging with course materials or having difficulty consistently performing poorly on assessments. In that case, educators can intervene early and provide personalized support. This can help students re-engage with course materials and improve their performance.
Enhancing Student Engagement
By providing personalized instruction and support, learning analytics can enhance student engagement. For instance, when students receive individualized support, they are more likely to be motivated and engaged in their coursework.
As a result, they are more likely to succeed academically. This approach creates an inclusive learning environment where every student has the opportunity to excel.
Unstructured Data Extraction: Turning Raw Data into Actionable Insights
In the age of e-learning and mobile learning, the amount of data generated by students and instructors is growing exponentially. However, most of this data is unstructured and lies dormant, leaving educators clueless to pursue new ways to extract valuable insights that can shape the learning journey for students.
What kind of unstructured data are we talking about? Consider sources such as student feedback, discussion forums, social media posts, and emails. Analyzing this data can provide deep insights into student preferences and learning outcomes.
Fortunately, AI and machine learning advancements have paved the way for institutes to unravel the valuable insights hidden in these unstructured data sources. Modern AI-powered data extraction tools can effectively process and analyze unstructured data.
Let’s look at some techniques that educators can use to guide the decision-making process:
- Text Mining: By using natural language processing (NLP) algorithms, text mining tools can analyze unstructured textual data, like discussion forums and student feedback, to identify patterns and areas of improvement. For example, NLP can be used to analyze student behavior and provide personalized recommendations for further study.
- Social Media Analysis: Social media platforms like Twitter and Instagram can provide valuable insights into student opinions and sentiments. With sentiment analysis tools, educators can analyze the tone and emotions expressed in social media posts. This will give them a better understanding of their students’ needs and experiences.
- LMS Data Analysis: Learning management systems generate vast amounts of data on student behavior, such as time spent on course materials, engagement with discussion forums, and quiz scores. Using data analytics tools, educators can identify patterns in student behavior, like areas of difficulty or low engagement, and adjust their teaching strategies accordingly.
- Predictive Analytics: Predictive analytics can forecast student performance based on historical data. By leveraging machine learning algorithms, educators can identify early warning signs of at-risk students. Consequently, they can provide targeted interventions to prevent students from falling behind.
Best Practices for Implementing Learning Analytics
Implementing learning analytics can be daunting, but the right approach can lead to improved student success and more personalized learning experiences. Here are three key best practices steps for incorporating learning analytics to enhance teaching and learning:
- Defining clear goals and objectives: Before implementing learning analytics, it’s crucial to identify the specific goals and objectives the institution wants to achieve. This ensures the data collected is relevant and helps achieve the desired outcomes. It’s also important to clearly communicate these goals to all stakeholders, including students, faculty, and administrators.
- Integrating data from multiple sources: Learning analytics involves collecting and analyzing data from various sources, such as student information systems, learning management systems, and social media. Integrating this data and ensuring its accuracy, completeness, consistency, and privacy is essential. This requires using a data integration platform to extract, transform, and load (ETL) data from different sources into a centralized repository.
- Ensuring data privacy and security: Protecting sensitive student information is crucial in learning analytics. Institutions must have clear policies and procedures for data collection, storage, and usage, including data anonymization and encryption. Data access should be limited to authorized personnel, and compliance with relevant data protection regulations such as GDPR and CCPA is essential.
Learning Analytics: Shaping the Future of Education
As we continue to navigate the ever-evolving landscape of education, it’s clear that AI-driven learning analytics will play a critical role in shaping the future of student success. By embracing these technologies and leveraging their potential, educators and institutions can empower learners to achieve their goals and reach their full potential.
But to fully harness the power of AI-driven learning analytics, it’s important to have a reliable data extraction tool like Astera ReportMiner. With its advanced data extraction capabilities, ReportMiner can help you easily extract and integrate data from various sources to create a unified student database.
Ready to unlock the potential of AI-driven learning analytics? Contact us now and take the first step towards a brighter future for education!