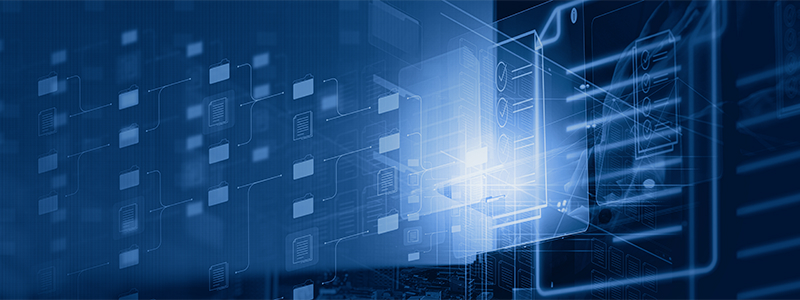
All You Need to Know About Data Completeness
Data completeness plays a pivotal role in the accuracy and reliability of insights derived from data, that ultimately guide strategic decision-making. This term encompasses having all the data, ensuring access to the right data in its entirety, to avoid biased or misinformed choices. Even a single missing or inaccurate data point can skew results, leading to misguided conclusions, potentially leading to losses or missed opportunities. This blog takes a deep dive into the concept of data completeness, exploring its importance, common challenges, and effective strategies to ensure that datasets are comprehensive and reliable.
What is Data Completeness?
Data completeness refers to the extent to which all necessary information is present in a dataset. It indicates whether there are any missing values or gaps in the data. When all relevant data points are included, a dataset is considered complete. In contrast, incomplete data contains missing or empty fields, which can hinder analysis and decision-making.
Examples of Incomplete Data
- Survey Data with Missing Responses
- Customer Database with Inconsistent Entries
- Financial Records with Incomplete Transactions
The Importance of Complete Data
When it comes to drawing conclusions and making informed decisions, data completeness matters more than businesses often realize. Data Completeness leads to:
- Improved Accuracy: Complete data ensures that analyses, models, and decisions are based on the most accurate representation of the situation. Incomplete data may lead to skewed results or erroneous conclusions.
- Increased Reliability: With complete data, findings and predictions gain higher reliability, minimizing the likelihood of errors stemming from data gaps and enhancing the trustworthiness of results.
- Optimized Decision-making: Complete data empowers decision-makers with the necessary information to make informed and timely decisions. It reduces uncertainty and enables stakeholders to assess risks and opportunities more accurately.
- Long-term Planning: Complete datasets support long-term planning efforts by providing reliable historical data, enabling organizations to identify trends and make informed projections for the future.
- Higher Customer Satisfaction: Complete data supports better understanding of customer needs and preferences, enabling organizations to tailor products, services, and experiences effectively.
The Role of Data Completeness in Data Quality
Completeness is one of the six primary dimensions of data quality assessment. Data quality is a broader term that encompasses various aspects of data, including completeness, accuracy, consistency, timeliness, and relevance, among others. It represents the overall condition of data and its fitness for use in a specific context or application. Data completeness, on the other hand, refers to the extent to which all required data elements or attributes are present and available in a dataset.
Data completeness is a measure that directly affects the accuracy and reliability of data. When important attributes or fields are missing, it can lead to erroneous analyses and incorrect conclusions. Incomplete data may also skew statistical measures, such as averages or correlations, potentially leading to flawed insights. Rather than engaging in the data quality vs. data completeness debate, it is crucial to recognize that prioritizing data completeness is fundamental for ensuring high data quality.
Data Completeness vs Data Accuracy vs Data Consistency
Understanding the differences between data completeness, data accuracy, and data consistency is crucial for ensuring the quality and reliability of data in any organization. Here’s a comparison table highlighting the differences between data completeness, data accuracy, and data consistency:
Aspect | Data Completeness | Data Accuracy | Data Consistency |
Definition | Presence of all required data elements or attributes in a dataset. | Correctness, precision, and reliability of data values. | Uniformity and conformity of data across different databases, systems, or applications. |
Focus | Ensures all expected data points are present without any missing values. | Ensures data values reflect real-world entities accurately and reliably. | Ensures data remains synchronized and coherent across various sources or systems. |
Concerns | Missing data points, gaps in datasets. | Errors, discrepancies, inconsistencies in data values. | Conflicts, contradictions, discrepancies between datasets or systems. |
Importance | Essential for comprehensive analysis and decision-making. | Critical for making informed decisions and accurate reporting. | Vital for reliable analysis, preventing errors, and ensuring trust in data. |
Example | Ensuring all sales transactions are recorded in a sales database. | Verifying that customer contact information is correctly entered in a CRM system. | Ensuring product prices are consistent across different sales channels. |
Mitigation | Implementing data validation checks, data collection protocols. | Data cleansing, verification against reliable sources. | Implementing data integration strategies, synchronization mechanisms. |
How To Determine and Measure Data Completeness
There are several approaches to assess data completeness, including attribute-level and record-level approaches, as well as techniques like data sampling and data profiling. Here’s an overview of each approach:
Attribute-level Approach
In the attribute-level approach, each individual data attribute or field within a dataset is examined to determine its completeness. To measure completeness at this level, users can calculate the percentage of non-null or non-missing values for each attribute. For categorical attributes, users may also look for the presence of all expected categories or values.
Example: A dataset contains customer information, including attributes like name, age, email, and phone number. To measure completeness at the attribute level, one would examine each attribute to see how many records have missing values. For instance, if 90% of the records have a value for the “age” attribute, but only 70% have an email address, the email attribute would be considered less complete.
Record-level Approach
In the record-level approach, entire records or rows of data are evaluated for completeness. This involves assessing whether each record contains all the necessary attributes or fields, and if those fields are populated with meaningful data. Completeness can be measured by calculating the percentage of fully populated records in the dataset.
Example: Continuing with the customer information dataset example, with the record-level approach, each record is assessed as a whole. If a record is missing any essential attribute (e.g., name or email), it would be considered incomplete. For instance, if 70% of records have non-null name and email, the dataset will be 70% complete.
Data Sampling
Data sampling involves selecting a subset of data from the larger dataset for analysis. Sampling can be random or stratified, depending on the characteristics of the dataset and the objectives of the analysis. By analyzing a sample of the data, you can infer the completeness of the entire dataset, assuming the sample is representative.
Example: Let’s say there’s a massive dataset with millions of records. Instead of analyzing the entire dataset, one might randomly sample 1,000 records and assess completeness within this sample. If the sample is representative of the overall dataset, findings can be extrapolated to estimate completeness across the entire dataset.
Data Profiling
Data profiling is a systematic analysis of the structure, content, and quality of a dataset. It involves examining various statistical properties of the data, such as distributions, frequencies, and summary statistics. Profiling can help identify frequency of missing values, outliers, duplicates, and other data quality issues that may affect completeness. Tools like histograms, summary statistics, frequency tables, and outlier detection algorithms can be used for data profiling.
Example: Using data profiling tools or techniques, one can generate summary statistics and visualizations to identify frequency of missing values across different attributes. For instance, a histogram could be generated showing the distribution of missing values for each attribute or calculating the percentage of missing values for each attribute.
5 Common Challenges in Ensuring Data Completeness
- Data Entry Errors: Human errors during data entry, such as typos, missing values, or incorrect formatting. Incomplete datasets may contain missing values due to various reasons, including equipment malfunctions, respondent non-response, or data collection errors.
- Data Integration Issues: Combining data from multiple sources can cause incompatibilities in data structures or identifiers, which can lead to incomplete or inconsistent datasets.
- Data Quality Control: Inadequate quality control processes can lead to incomplete data, as errors may go undetected during data collection or processing.
- Lack of Data Governance: Absence of clear data governance policies and procedures can result in inconsistent data definitions, ownership issues, and poor data management practices, ultimately leading to incomplete datasets.
- Obsolete Data Systems and Architectures: Inadequate infrastructure or outdated technologies may hinder data collection, processing, and storage. Incomplete data sets can also be due to data privacy regulations and compliance requirements which may limit access to certain data.
Strategies to Ensure Data Completeness
Establish Clear Data Entry Protocols: Organizations should develop clear guidelines and protocols for data entry to ensure consistency and accuracy. This includes defining data fields, formats, and validation rules to minimize errors during data entry.
Implement Data Validation Checks: Automated data validation checks should be implemented to identify incomplete or inaccurate data entries in real-time. This can include range checks, format checks, and cross-field validations to ensure data accuracy and completeness.
Regular Data Audits: Conducting regular audits of the data can help identify incomplete or missing data points. These audits should involve comparing the dataset against predefined standards or benchmarks to ensure completeness and accuracy.
Use Data Profiling Tools: Data profiling tools can access the contents of a dataset, providing statistics such as minimum and maximum values, unique value count, missing value count etc. By leveraging these tools, organizations can proactively address data completeness issues and take corrective actions.
Implement Data Quality Monitoring: Establishing a robust data quality monitoring process allows organizations to continuously monitor the completeness of their data. Alerts and notifications can be set up to flag any deviations from expected data completeness levels.
Incorporate Data Governance Policies: Implementing data governance policies ensures that data completeness requirements are clearly defined and enforced across the organization. This includes assigning responsibilities for data stewardship and establishing processes for data quality management.
Data Enrichment Strategies: In cases where data completeness is compromised, organizations can employ data enrichment techniques to fill in missing data points. This may involve integrating external data sources or using algorithms to extrapolate missing values based on existing data.
Using Automated Tools for Complete Data
Automated tools play a crucial role in ensuring the completeness and reliability of data across various domains. These tools facilitate the collection, processing, and analysis of large datasets efficiently, enabling organizations to derive valuable insights and make informed decisions. By automating tasks such as data cleaning, integration, and analysis, these tools streamline workflows and minimize errors, resulting in more accurate and actionable information.
Additionally, automated data visualization enables stakeholders to understand complex patterns and trends quickly, facilitating communication and decision-making processes. Moreover, automated tools help organizations maintain data security and compliance with regulations, mitigating risks associated with data handling.
Astera: Ensuring Data Completeness with Advanced No-Code Data Management
Astera offers an end-to-end no-code data management platform equipped with advanced and automated capabilities for data integration, extraction, and preparation. With a wide range of features, Astera empowers users to create and maintain automated data pipelines that deliver accurate and timely data.
With Astera, users can seamlessly extract and cleanse data from unstructured sources, leveraging AI-powered document processing capabilities. Users can effortlessly integrate data from diverse file sources and database providers, supported by a data pipeline builder that accommodates various formats, systems, and transfer protocols. This reduces the challenge of incompatibilities in data structures or identifiers, which often lead to incomplete or inconsistent datasets.
Through the Astera Dataprep feature, users can cleanse, transform, and validate extracted data with point-and-click navigation, supported by a rich set of transformations including join, union, lookup, and aggregation. With attributes like active profiling, data quality rules, and preview-centric grids, Astera ensures data cleanliness, uniqueness, and completeness, providing users with attribute-level profile and vivid graphical representations to easily identify patterns of completeness or lack thereof.
Astera also offers ease of integration, allowing users to effortlessly utilize cleaned and transformed data in analytics platforms, thus enabling informed decision-making based on comprehensive and reliable data.
Achieve data completeness effortlessly with Astera today – Book a personalized demo now!