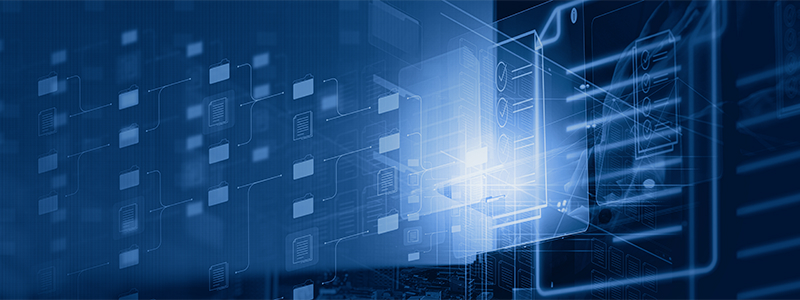
Exploring the Connection Between Data Governance and Data Quality
Data Governance vs. Data Quality
Data governance and data quality are closely related, but different concepts. The major difference lies in their respective objectives within an organization’s data management framework. Data quality is primarily concerned with the data’s condition. It ensures the data is complete, accurate, reliable, and consistent.
On the other hand, data governance refers to the overall management, maintaining compliance, and ensuring the security of data assets within an organization. Data governance sets the framework and guidelines under which data quality initiatives operate. Both data governance and quality are equally important for an organization to derive value from their data and make informed decisions.
Understanding Data Quality
Data quality measures how much data sets meet the specific requirements and expectations of their intended use, ensuring that decisions based on good quality data are rational and defensible. The significance of data quality stems from its role in decision-making. High-quality data allows organizations to understand their customers and identify new market trends. It ensures that:
- Marketing campaigns reach the right audience.
- Financial forecasts are reliable.
- Customer service is effective and personalized.
Achieving high data quality is not a one-time activity but an ongoing, iterative process, called data quality management. It involves a series of steps, from data acquisition through storage and management to its final use. At each stage, specific measures must be taken to ensure data integrity. For example, data validation rules can be applied during collection to prevent errors, while regular audits can identify inconsistencies or outdated information that may affect data reliability.
Understanding Data Governance
Data governance is a thorough framework that ensures data is accurate, accessible, consistent, and protected, thereby supporting the organization’s objectives. Take data governance as a rulebook for effective data management, establishing protocols for the accurate collection, storage, and utilization of data.
Data governance helps a company achieve its goals by providing a well-structured framework and setting clear and well-communicated policies that give all employees a common understanding of how data should be handled. This structure also defines the responsibilities of different roles within the organization, such as who is accountable for maintaining the accuracy of data and who has the authority to access sensitive information.
The sensitivity of personal data and growing privacy concerns make data governance all the more important for organizations. In 2022, there were 1,774 instances of organizational data breaches, affecting over 392 million individuals globally. These incidents exposed legally protected personal information of people utilizing services ranging from financial and healthcare to social services.
Exploring the Differences Between Data Governance and Data Quality
Data Quality | Data Governance | |
Objective | To ensure that the data is standardized and free of inconsistencies and errors. | To ensure effective management, protection, compliance and utilization of organizational data. |
Techniques | Data profiling, cleansing, standardization, enrichment, and validation. | Data lineage, access controls, policy development, data stewardship, data cataloging and metadata management. |
Tools | Data quality tools, specialized databases designed to support data quality tasks. | Data governance platforms such as Astera and meta data management tools. |
Outcome | Trustworthy data that can be used reliably for decision making. | A controlled data environment, having clear data policies and improved data management practices. |
Where Data Governance and Data Quality Overlap
The overlap between data governance and data quality lies in their mutual goal of ensuring that data is a reliable asset for the enterprise. Data governance determines who needs to do what with which data under specific conditions and identifies procedures and tools to enhance data quality. Data quality ensures that the data is accurate, complete, and valuable within this system.
The connection between data quality and data governance comes from shared standards, metrics, and responsibilities of data roles. It helps create a symbiotic relationship that enhances the overall value of an organization’s data:
- Framework and Execution: Data governance provides the framework and policies for data management, while data quality focuses on executing these policies. For instance, data governance might establish a policy that all data should be consistent and accurate. Data quality measures like data cleaning and validation, would then be used to ensure that this policy is effectively implemented.
- Enhancing Trust: Data governance and data quality work together to enhance trust in data. Data governance ensures clear policies and procedures for data management, which can increase confidence in the data. Meanwhile, data quality measures ensure that the data is accurate, reliable, and relevant, further enhancing trust.
- Risk Management: Data governance sets the rules for compliance with regulations and standards, while data quality ensures that the data adheres to these rules. This complementary relationship is crucial for risk management, as it helps to prevent breaches of regulations and standards, which can result in penalties and damage to the organization’s reputation.
- Decision-Making: High-quality data is essential for informed decision-making. Data governance ensures that the right data is available to the right people at the right time, while data quality ensures that this data is accurate and reliable. Together, they enable organizations to make decisions based on trustworthy data.
Data cataloging is another area where data quality and governance overlap. From the governance perspective, a data catalog provides a holistic picture of what data exists and who’s responsible for it—allowing for setting data standards and access controls. On the data quality side, the catalog helps identify potential issues through data profiling and lineage tracking, which enables data stewards to implement processes for improving data accuracy and consistency.
6 Steps to Integrate Data Quality within a Data Governance Framework
Integrating data quality within a data governance framework is a strategic initiative that significantly enhances the value of an organization’s data. Such integration ensures that data is well-managed but also accurate, reliable, and useful for decision-making. Here are some steps to effectively integrate data quality within a data governance framework:
- Define Data Quality Goals
Begin by outlining what high-quality data means for your organization. This step generally includes accuracy, completeness, consistency, timeliness, and relevance. It is important to align these goals with the organization’s overall objectives and communicate them clearly to all stakeholders.
- Establish Data Quality Metrics
After defining the goals for data quality, establish metrics to measure data health. Data quality metrics could include error rates, completeness percentages, or consistency scores. These quantitative measures will assess data quality and track improvements over time.
- Incorporate Data Quality into Policies & Procedures
All data governance policies and procedures, including those for data collection, storage, processing, and usage, should prioritize data quality as a critical consideration. Incorporating data quality into these procedures ensures its maintenance throughout the data lifecycle.
- Assign Data Quality Responsibilities
Integrating data governance and data quality calls for assigning responsibilities for maintaining data health. For example, designating a data steward to define and enforce data quality standards for their assigned data, or establishing data quality roles within the IT department to focus on data profiling, data cleansing, and implementing data quality checks.
- Implement Data Quality Tools & Techniques
The use of data quality tools and techniques is crucial in improving the quality of data. Modern data quality tools combine data standardization, validation, profiling, and cleansing, along with several other features, in a single package. Automating data quality tasks with these tools simplifies data quality management.
- Monitor & Improve Data Quality
The final step is to monitor data quality using the established metrics continuously. Regular monitoring identifies any issues or trends and enables corrective action as needed. Incorporating data quality within a data governance framework allows organizations to ensure their data is well-managed, accurate, reliable, and valuable.
Conclusion
Data governance and data quality are closely linked, influencing the reliability and usability of data. Despite their differences in scope and application, data quality and data governance create a unified approach to data management, which allows companies to maximize the benefits of their data assets. Building on this, Astera offers an effective solution that simplifies sharing and managing data across a company through a single platform.
Astera’s data management platform ensures data quality and trustworthiness by providing the percentage of data health based on defined data quality rules. It enhances organizational decision-making by automatically correcting errors, detecting inaccuracies, and standardizing data. Data governance contributes by organizing data assets, managing data access, and making data trustable and understandable.
If you’re looking to enhance your decision-making process with easier access to data, consider book a demo now!
Ready to boost your decision-making with high-quality, trustworthy data?
Try Astera’s data governance platform to see how you can improve data health, correct errors, and standardize your data effortlessly.
Start you 14-days-trial now!