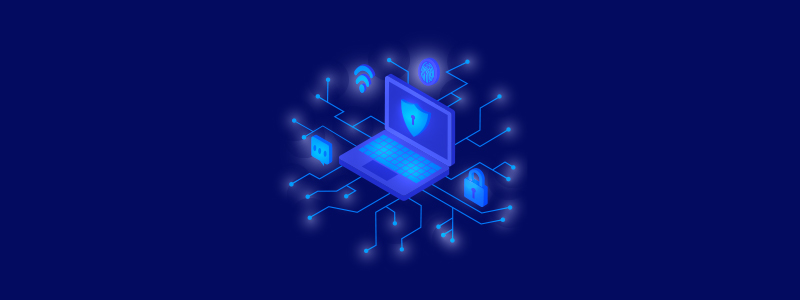
Data Integrity vs. Data Quality: Here’s How They Are Different
Data Integrity vs. Data Quality in a Nutshell
Data integrity refers to protecting data from anything that can harm or corrupt it, whereas data quality checks if the data is helpful for its intended purpose. Data quality is a subset of data integrity. One can have accurate, consistent, and error-free data, but it is only helpful once we have the supporting information for this data.
Data integrity and quality are sometimes used interchangeably in data management, but they have different implications and distinct roles in enhancing data usability.
The Importance of Data Quality and Data Integrity
Data serves as the lifeblood of organizations, supporting every initiative from product development to marketing campaigns. The success of these decisions relies on the quality and trustworthiness of data, making data quality and integrity foundational to success.
Data Quality: Empowering Informed Decision Making
Data quality measures how well data meets requirements and fits the intended purpose. Experts usually assess it using various criteria, whose importance may vary based on the specific data, stakeholders, or intended use.
- Improved Decision Making
Reliable analytics and insights depend on high-quality data. Data quality allows marketing campaigns to target audiences precisely. It also aligns product development with customer needs and supports data-backed operational improvements for maximum efficiency.
- Improved/Enhanced Customer Experience
Organizations use complete and accurate customer data to personalize interactions through various platforms, like social media, websites, etc. High-quality data also helps anticipate the consumer’s needs and can identify issues swiftly to resolve them. This approach fosters customer loyalty and satisfaction, enhancing the brand’s perception.
- Optimized Operations
High-quality data is a single source of truth, removing inconsistencies and discrepancies to prevent wasted effort. It streamlines workflows, reduces errors, and lessens the need for rework. As a result, productivity rises, costs drop, and overall efficiency improves.
Data Integrity: Building Trust
Data integrity concerns the inherent quality of data and aims to maintain this quality throughout the data lifecycle. This action includes all stages, from creation and storage to processing and analysis, ensuring the data remains accurate and consistent.
- Data security
Security ensures that data remains protected from unauthorized access, modification, or deletion. Access controls, encryption, and intrusion detection systems prevent unauthorized individuals from altering or tampering with the data. Data security creates trust among partners and stakeholders and strengthens the organization’s reputation.
- Data lineage
Data lineage tracks the origin and transformation of data. Lineage tracking upholds data integrity by keeping a clear audit trail of modifications and identifying the source and reason for each change.
- Auditability
Auditing capabilities enable tracing changes to the data and identifying who made them. Logging all data modifications, including the time, responsible user, and nature of the change, reinforces data integrity. This process fosters transparency and accountability, which are crucial for building trust in the data.
The Mutual Relationship
Data quality and data integrity work together to enhance data usability. High-quality data becomes worthless if its integrity is not secure. Similarly, maintaining data integrity does not serve its purpose if the data is inaccurate or incomplete.
For example, if we have a customer database filled with verified, complete information showing high data quality. However, if a system flaw allows unauthorized changes (indicating low data integrity), the entire database’s reliability is at risk.
Data Integrity vs. Data Quality: Examples and Applications
It’s evident that data quality and integrity are closely related, but understanding the subtle differences is essential to maximize the data’s value.
Financial Reporting
Consider a company preparing its quarterly financial report. Data integrity plays an important role in maintaining the accuracy and security of financial data.
- Data Security: Access controls restrict unauthorized users from modifying financial figures, safeguarding data integrity.
- Data Lineage: The company tracks the origin of revenue and cost data, ensuring it hasn’t been tampered with during its journey from sales figures to the final report.
- Auditability: Every modification made to the data, such as adjustments or corrections, is logged with timestamps and usernames. This audit trail allows for verification and ensures no fraudulent alteration of the data.
Here, data integrity guarantees the financial report reflects the true state of the company’s finances, fostering trust with investors and stakeholders.
Customer Segmentation
Let’s consider a marketing team segmenting customers for a targeted email campaign. Here, data quality takes center stage:
- Accuracy: Customer email addresses must be accurate to ensure successful campaign delivery. Incorrect data (e.g., typos) would make the segmentation exercise futile.
- Completeness: Complete customer profiles, including purchase history and demographics, are crucial for effective segmentation. Missing data would limit the ability to create targeted customer groups.
- Consistency: Customer names and addresses should be formatted consistently across the database. Inconsistencies (e.g., variations in capitalization) can lead to duplicate entries and skewed results.
How to Ensure Data Quality and Integrity
Maintaining high data quality and data integrity requires a comprehensive data quality management strategy.
Measures to Ensure Data Quality
- Data profiling: Data profiling helps pinpoint areas requiring improvement by identifying missing data, inconsistencies, outliers, and duplicate records. Regularly analyze data to determine such anomalies.
- Data cleansing: Implement processes to correct errors, remove duplicates, and ensure consistent formatting throughout the data set. Data cleansing involves using data cleaning tools and establishing clear data entry guidelines.
- Data Standardization: Data standardization transforms data into a format that computers can read and understand. By standardizing data, detecting errors and ensuring accuracy becomes much easier. This accuracy is essential for providing decision-makers with reliable and precise information.
- Data validation: Enforce data validation rules at the point of entry to prevent inaccurate or invalid data from reaching your destination systems. Validation includes defining acceptable value ranges, mandatory fields, and data type restrictions.
- Data quality metrics: Data quality metrics are tools to measure and improve data quality. Organizations can ensure they possess high-quality data by selecting and applying the appropriate metrics to evaluate the data. Data quality metrics include timeliness, completeness, accuracy, validity, duplication, and uniqueness.
- Data governance framework: Establish a data governance framework outlining data quality standards, ownership, and accountability. The framework should also define data quality metrics and procedures for monitoring and improving data health.
- Data lineage tracking: Implement data lineage tracking tools to understand the origin and transformations of data throughout its lifecycle. Data lineage tracking allows for tracing any potential issues back to their source.
Measures to Ensure Data Integrity
- Remove duplicate data: Duplicate data creates ambiguity, leading to errors and breaches in data integrity. Large organizations employ dedicated teams to clean duplicate files. Whether to choose a team or utilize software to remove duplicates depends on the data volume or size of the organization.
- Access controls: Lacking effective access controls in an organization increases the risk to data integrity. Implementing the principle of least privilege is one of the most effective strategies. It only restricts access to essential users, maintaining strict control and preserving data integrity.
- Keep an audit trail: Audit trails give organizations clues to pinpoint the problem’s source for effective resolution as they record all system data, including database or file changes. They must be tamper-proof, preventing user manipulation. These trails should generate automatically, track every database and file event, link events to the users involved, and include timestamps for all occurrences. Regularly auditing these trails is a best practice to uncover weaknesses or areas for improvement and enhance data integrity.
- Data encryption: Data encryption protects data integrity within an organization by keeping it confidential. This security measure safeguards data during rest, i.e., when stored in a database and during transmission, for example, when moving to another database.
- Backup the data: To ensure data integrity, organizations should adopt a two-pronged approach. First, implement regular data backups to safeguard the information against potential losses from hardware malfunctions or cyberattacks. Secondly, establish a data recovery plan to enable the accurate restoration of data in accidental deletion or corruption cases.
Data Integrity vs. Data Quality
Feature | Data Quality | Data Integrity |
Focus | Inherent characteristics of the data itself | Maintaining the trustworthiness of data throughout its lifecycle |
Objective | Ensures data is fit for its intended purpose | Ensures data remains accurate, reliable, and unaltered |
Key Attributes | Accuracy, Completeness, Consistency, Validity, Timeliness | Security, Lineage, Auditability |
Impact | Affects data analysis, decision-making, and operational efficiency | Affects, compliance, and risk management |
Mechanism | Data cleansing tools, data validation rules, data governance framework | Encryption, access controls, audit trails, data backup and recovery |
Concluding Thoughts
Data quality and data integrity are distinctive concepts but not mutually exclusive. A comprehensive data management strategy considers both enhancing data analyses and business decisions. Automated data management tools with built-in features to address data quality and integrity issues help organizations ensure their business decisions rely on healthy data.
Astera provides a unified data management solution that helps you ensure data quality and integrity. With Astera, you can automate data cleansing, profiling, and validation tasks while leveraging built-in data governance features, such as data discovery, data quality rules, and data ownership—all within a single, no-code, user-friendly platform.
Schedule a demo or download a free 14 day trial to experience Astera’s data management solution and improve your organizational data quality and integrity.
Discover how Astera's solutions can elevate your data quality and integrity. Schedule a demo today to see how easy and efficient data management can be with our comprehensive, no-code platform. Discover how Astera's data management solutions can elevate your data quality and integrity. Schedule a demo or start your free trial today to see how easy and efficient data management can be with our comprehensive, no-code platform. Trust your data's accuracy and security with Astera.Enhance Your Data Quality and Integrity with Astera