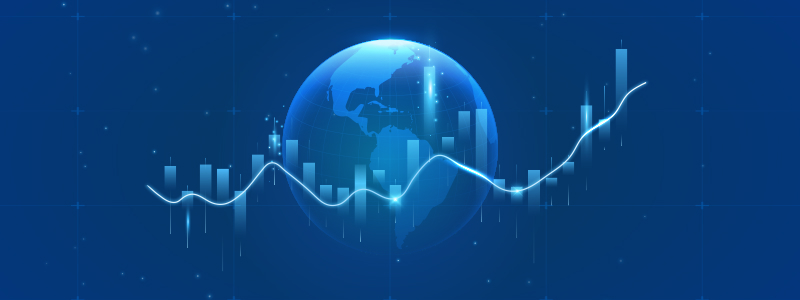
Enterprise AI Strategy: Why AI Agents Should Be Your First Step
Since generative artificial intelligence (GenAI) captured mainstream attention a few years ago, businesses have been looking for ways to implement AI into their operations. There are some obvious reasons for this shift: saved time, increased productivity, and decreased need for manual effort. But there’s also another factor at play—the realization that not embracing AI now means getting left behind by the competition.
When faced with pressure to become an AI-first business, starting with large language model (LLM) applications or experimenting with predictive analytics can be tempting. However, there are compelling reasons why AI agents should be the priority while developing an enterprise AI strategy.
This blog explores why starting with AI agents makes sense, and how you can implement these agents successfully. Let’s begin.
Enterprise AI and Creating a Strategy That Works
Enterprise AI is the strategic integration of artificial intelligence across all business functions to automate processes, improve decision-making, and unlock new value streams. An enterprise AI strategy is a structured plan detailing how your organization will leverage AI to achieve specific, measurable goals.
Developing a robust AI strategy is crucial, as it sets the foundation for all subsequent AI initiatives. This strategy must clearly define the goals you hope to achieve. However, many organizations struggle to move from AI aspirations to tangible results.
To bridge this gap, your strategy needs to be both clear and actionable, with a focus on achievable milestones, effective resource allocation, and continuous alignment with overarching business goals. Indeed, a highly effective way to translate an enterprise AI strategy into tangible results is by deploying autonomous AI agents to automate targeted tasks and deliver measurable business value.
This approach aligns with the principle that a successful enterprise AI strategy doesn’t start with complex, high-risk projects or speculative AI investments. Instead, it begins by identifying areas where AI can provide immediate, measurable value. This ensures that the integration of AI is manageable, less risky, and capable of producing quick wins that justify further investments.
Deploying AI agents to automate targeted tasks and deliver measurable business value is a highly effective way to operationalize an enterprise AI strategy once a solid data foundation and governance framework are in place.
What is an AI Agent?
AI agents are advanced artificial intelligence systems that can be configured using LLMs to understand and complete tasks independently, make decisions, and adapt to the environment that they operate in with minimal human intervention.
“Think of agents as the new apps for an AI-powered world,”
– Jared Spataro, Chief Marketing Officer, AI at Work (Microsoft)
Why Prioritize AI Agents in Your Enterprise AI Strategy?
1. AI Agents Deliver Immediate ROI
AI agents can generate returns quickly since they eliminate routine human effort, which is the biggest obstacle in most business processes.
When you deploy AI agents to perform data entry, oversee scheduling and reporting, handle document processing, or undertake basic support functions, you can quickly reallocate your human talent to higher-value activities.
Put simply, each human hour that’s not spent on routine work amounts to immediate cost savings and productivity gains. Unlike speculative AI investments, you can measure agent ROI directly through tangible operational metrics.
2. AI Agents Lay The Foundation for More Advanced AI
Starting with AI agents creates infrastructure you can use for future AI success.
- The data pipelines you develop for agents can become the foundation for future AI initiatives.
- The integration patterns you establish when deploying agents can become templates for further AI integration.
- The monitoring frameworks you create for agents can grow into broader AI observability.
- Feedback mechanisms for agent improvement can become the prototype for future AI feedback loops.
The governance approaches you create for agents can evolve into enterprise AI governance.
3. Agents Add to Your Organizational AI Capabilities
Deploying AI agents improves the human skills necessary for long-term AI success.
- Cross-functional teams practice effective collaboration on AI initiatives in the lower-risk context of agent deployment.
- Technical teams gain valuable practical experience with prompt engineering, context management, and AI guardrails.
- Business teams develop more realistic expectations about AI’s potential and limitations.
- Leadership gains experience assessing AI investments and managing AI-specific risks.
The organization builds frameworks for AI-driven process redesigns that future initiatives can reuse.
4. Agents Provide Cultural Acclimatization to AI
- The productivity benefits of agents increase your employees’ receptiveness to further AI capabilities.
- Collaborative human-agent workflows can become the template for future human-AI interactions.
Your organization develops new performance expectations and management processes that are tailored to an AI-enhanced workforce.
5. Agents Enable Experiential Learning Through Rapid Iteration
- AI agents are quickly deployable and continuously improvable, unlike bigger AI projects that can take months to develop.
- AI agents are built from independent, reusable components that you can combine in different ways for different tasks. This modular approach enables controlled experimentation in a variety of contexts and processes.
- Business users can directly influence agent behavior through feedback and preference input, creating tighter learning loops.
You Know Why AI Agents Should Be a Priority. Here's How to Build Them.
Astera offers pre-built templates, a variety of functions, and a visual, drag-and-drop designer for fast & easy AI agent development. Build, deploy, and see your AI agents in action for yourself.
Speak to Our TeamTypes of AI Agents to Consider First
When focusing on AI agents in your enterprise AI strategy, consider the following high-impact, low-risk applications:
1. Process Automation Agents
These agents can handle repetitive business processes from start to finish, including:
- Invoice processing and accounts payable
- Employee onboarding documentation
- Compliance reporting
- Data migration and cleansing
2. Knowledge Workers’ Assistants
These agents support knowledge workers by:
- Summarizing documents and meetings
- Drafting everyday communications
- Collecting and organizing information
- Managing calendars, scheduling, and priorities
3. Customer Interaction Agents
These agents improve customer experience by offering:
- Personalized support for common issues
- Guided product discovery
- Order status tracking and updates
- Post-purchase follow-ups
4. Data Management Agents
These agents can improve data quality and accessibility by:
- Monitoring data quality and flagging anomalies
- Enriching data with additional context
- Creating custom reports and visualizations
- Managing access permissions
AI Agent Implementation: A Step-by-Step Guide
Step 1: Start with Process Discovery and Prioritization
Identify processes that are high-volume and repetitive, rule-based with clear distinction criteria, currently causing bottlenecks or delays, and don’t require complex judgment or creativity.
Once identified, prioritize these processes based on their potential business impact, implementation complexity, and organizational readiness.
Step 2: Aim High, Shoot Low
Next, begin developing targeted pilot projects for your AI agents that meet the following criteria:
- Can be implemented quickly
- Have clearly defined metrics for success
- Impact a small but meaningful user group
- Can be scaled if successful
Design these pilot projects while allowing for future expansion. You’ll start small but ensure that the underlying architecture can support broader deployment.
Step 3: Center Human-Agent Collaboration
Human-agent collaboration should be at the center of your efforts. Design implementations that can enhance human capabilities instead of replacing them.
Define clear handoff points between agents and humans, set up oversight mechanisms for agent activities, create feedback loops, and empower your employees to direct and customize agent behaviors. Such a collaborative approach can mitigate resistance and lead to improved outcomes.
Step 4: Create a Dedicated AI Team
Establish a dedicated AI team whose responsibilities include evaluating agent technologies, creating deployment standards, developing and sharing best practices, and managing the agent deployment roadmap.
Over time, this team can become the driving force behind organizational AI governance as your enterprise AI strategy evolves.
Common AI Implementation Challenges and How to Resolve Them
Challenge 1: Managing Organizational Change
The human component of AI implementation can often be more complex and trickier to navigate than its technical counterpart. Success depends heavily on helping employees navigate workflow changes, addressing their concerns, and encouraging the development of new skills.
Solution: Implement a comprehensive change management strategy with collaborative design workshops and transparent communication about how agents enhance human roles. Deploy in measured phases with feedback mechanisms, provide targeted training, and celebrate early wins to build momentum and demonstrate tangible benefits.
Challenge 2: Creating Effective Governance Frameworks
Even simple AI agents require well-thought-out governance structures to mitigate potential risks related to ethical and legal compliance, security and data privacy, accountability and explainability, and hallucinations and misinformation.
Solution: Develop a governance framework with data privacy protocols and regular audits, transparent documentation of decision processes, clear ethical boundaries, cross-functional oversight, and periodic reviews to adapt as capabilities evolve.
Challenge 3: Maintaining Business Value Focus
The excitement of advanced AI capabilities and their anticipated potential can often overshadow your AI strategy’s core purpose of addressing specific business needs.
Solution: Keep business outcomes at the forefront by defining success metrics tied to objectives, prioritizing high-impact use cases regardless of technical complexity, conducting regular value assessments, establishing stakeholder feedback loops, and celebrating results in business rather than technical terms.
Challenge 4: Accounting for Enterprise Scale
Initial successful deployments can create momentum that requires thoughtful consideration and planning to replicate at the enterprise level.
Solution: Prepare for scaling with modular architectures supporting enterprise-wide deployment, infrastructure roadmaps anticipating growth, flexible governance frameworks, knowledge-sharing communities of practice, and reusable components that accelerate future implementations.
High-Quality Data: The Not-So-Secret Ingredient for Successful AI Agents
High-quality data is the foundation of successful AI agents, directly impacting their accuracy, adaptability, and long-term viability. While cutting-edge algorithms drive AI development, the quality of the underlying data determines real-world performance. Research shows that 85% of all AI projects/models can fail due to subpar data quality or lack of relevant data.
A strong enterprise AI strategy should include a well-defined approach to data governance, ensuring that AI agents are trained on accurate, complete, and representative datasets. Well-curated training data allows AI models to generalize across diverse scenarios, improving their predictive accuracy and reducing bias-related risks.
As part of a structured enterprise AI strategy, organizations can establish data pipelines that support continuous learning, ensuring their AI agents stay responsive to changing conditions.
High-quality data also enhances efficiency, reducing the need for extensive data preprocessing and model retraining. This translates into cost savings, faster deployment, and better scalability for AI-driven initiatives.
Ultimately, businesses that treat data as a strategic asset rather than an afterthought are better positioned to develop AI agents that drive real value, improve user trust, and align with ethical AI standards.
Build Your AI Agents at Breakfast and Start Seeing Results by Lunch
With Astera, designing, building, and deploying high-impact, powerful AI agents is as easy as it gets. Use our visual designer, drag-and-drop objects, and pre-built templates, and have AI agents built using your business data ready to go within hours.
Start HereSumming It Up
The enterprise AI race will be won by organizations that execute strategically, starting with initiatives that deliver immediate value while building the foundation for future transformation.
AI agents represent the ideal first step in this journey. They deliver immediate ROI, establish essential technical and organizational foundations, facilitate cultural adaptation, and create accelerated learning cycles that inform future AI investments.
As you develop your enterprise AI strategy, make agents your first priority—not just one option among many, but the demonstrably superior path to successful AI transformation.
Astera AI Agent Builder: Your Data, Your Agents
Astera AI Agent Builder provides an AI-powered solution that lets you ideate, build, deploy, and refine autonomous AI agents—all within an intuitive, drag-and-drop visual interface. Use our award-winning unified ETL platform to integrate data from different sources, then clean, prepare, and connect it directly to your AI agents.
Astera AI Agent Builder accelerates AI adoption so that everyone in your organization can quickly create enterprise-ready agents.
Discover Astera AI Agent Builder’s powerful features or contact us for more information.