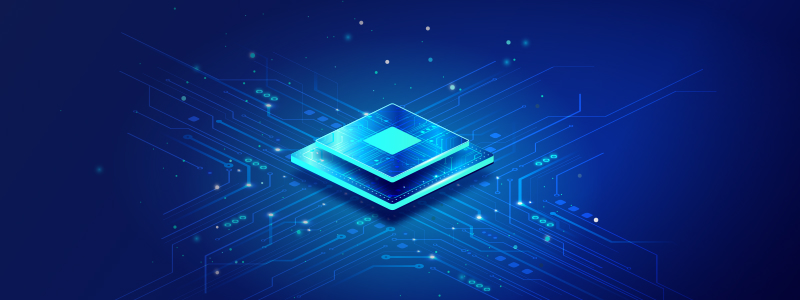
What is a Multi Agent System? Types, Application and Benefits
AI has evolved from simple rule-based systems to models capable of understanding language, generating images, and even assisting in complex decision-making. Yet, most AI systems still operate as a single, standalone entity. But what if AI could work like a team, where each agent brings its own strengths to the table? Multi-agent systems (MAS) make this possible by enabling real-time interaction and coordination among intelligent agents.
This shift redefines how AI interacts with the world, enabling smarter automation and more dynamic problem-solving.
What is a Multi Agent System?
A multi agent system comprises multiple independent entities, called agents, that work together to achieve a common goal. These agents can be software programs, robots, or even intelligent sensors, and they operate in a shared environment.
Each agent in a multi-agent system has three core characteristics:
- Autonomy: Each entity makes independent decisions based on its environment and objectives.
- Interactivity: They communicate and exchange information to improve decision-making and optimize performance.
- Adaptability: The system responds dynamically to new inputs, adjusting actions in real time to evolving conditions.
The Key Components of Multi Agent Systems
The building blocks of a multi-agent system define how agents interact, make decisions, and operate within an environment. They are:
- Large Language Models (LLMs)
LLMs serve as the knowledge base and reasoning engine in many modern multi-agent systems. These models help agents interpret data, recognize patterns, and make informed decisions. They enable natural language understanding, allowing AI agents to communicate effectively with users and other agents. LLMs also enhance problem-solving capabilities by analyzing vast amounts of information, learning from previous interactions, and improving their performance over time.
- Agents
Agents are the core functional units of a multi-agent system. Each agent operates with a specific goal, making independent decisions while interacting with other agents or external systems. Some agents follow predefined rules, while AI agents adapt to changing environments. Their ability to work individually or collaboratively makes them essential for automating complex workflows.
- Tools
Tools provide agents with specialized functionalities that extend their capabilities. These can include APIs, data retrieval modules, computational models, and external software integrations. Tools help agents perform domain-specific tasks more efficiently, such as retrieving real-time information, executing calculations, or processing images and text.
- Processes
Processes define the structure and rules governing agent interactions. They establish communication protocols, decision-making workflows, and conflict-resolution mechanisms. Well-defined processes ensure that agents collaborate effectively, share information efficiently, and execute tasks in an organized manner.
How Multi Agent Systems Work
Multi agent systems operate based on interactions between intelligent entities, their environment, and coordinated decision-making. Here’s how they function:
- Perception & Sensing – Agents collect data from their environment using sensors or inputs.
- Decision-Making – Based on the collected data, agents use rules, AI, or machine learning to determine their next actions.
- Communication & Coordination – Agents exchange messages, negotiate, or synchronize actions to achieve system-wide goals.
- Action Execution – Each agent performs tasks based on its decision-making process, influencing the system’s overall behavior.
- Learning & Adaptation – Some agents use learning techniques to improve performance and refine strategies over time.
Comparing Single Agent and Multi Agent Systems
A single agent system consists of one autonomous entity that operates independently to complete a task. It makes decisions based on its own data and environment without needing to communicate with other agents. These systems are simple, efficient for straightforward tasks, and easy to manage, but they lack coordination and adaptability in complex environments.
In contrast, a multi agent system involves multiple autonomous entities that interact, communicate, and collaborate to work towards a shared objective. These agents can either work cooperatively, competitively or in a hybrid manner. A multi agent system is particularly useful in dynamic environments where distributed intelligence and coordination improve efficiency.
Take traffic management as an example. In a single agent system, each traffic light operates on a fixed timer. It turns red, yellow, or green based on a set schedule, without considering real-time traffic conditions. Even if there’s no traffic on one side, the light still follows its programmed cycle, sometimes causing unnecessary delays.
On the other hand, in a multi agent system, traffic lights communicate with each other and adjust based on real-time conditions. Sensors detect vehicle flow, cameras monitor congestion, and nearby intersections exchange information to optimize traffic movement. If one road is congested, the system can extend the green light duration or reroute vehicles to reduce wait times.
Aspect | Single Agent System | Multi Agent System |
Operation | Works independently based on predefined rules. | Multiple agents collaborate and coordinate actions. |
Decision-making | Each agent makes decisions on its own. | Agents share information and make collective decisions. |
Adaptability | Limited flexibility; follows a fixed pattern. | Highly adaptable; adjusts based on real-time conditions. |
Efficiency | May struggle in complex environments. | Optimizes processes through coordination. |
Scalability | Difficult to scale as complexity grows. | Easily scalable by adding more agents. |
Communication | No interaction with other agents. | Agents communicate and share data. |
Fault Tolerance | Failure of one agent may impact the system. | More resilient—other agents can compensate for failures. |
Complexity | Simpler to develop and manage. | More complex due to coordination. |
Use Cases | Basic automation, rule-based tasks. | AI-driven systems, robotics, distributed networks. |
Types of Multi Agent Systems
Multi agent systems can be classified into different types based on how agents interact, collaborate, and function. Here are the main types:
Cooperative Multi Agent Systems
In cooperative multi-agent systems, agents work together to achieve a shared goal. They communicate, share information, and coordinate their actions to improve overall system performance. These systems are designed to enhance efficiency and problem-solving by leveraging collective intelligence.
Example: A fleet of autonomous delivery robots operates in a city. Each robot shares real-time traffic and route data with the others, helping to distribute delivery tasks efficiently and avoid congestion.
Collaborative Multi Agent Systems
Collaborative multi-agent systems involve agents that work together by communicating, sharing tasks, and coordinating actions to achieve a common goal. This setup allows agents to optimize each other’s performance, ensuring smoother workflows and more efficient execution of complex processes.
Example: A smart factory utilizes different types of robots to streamline production. Assembly robots construct products, quality-control robots inspect for defects, and packaging robots prepare items for shipping.
Mixed-Agent Multi Agent Systems
A mixed-agent system includes both cooperative and competitive agents within the same environment. Some agents may work together to achieve a common goal, while others act independently or compete for resources. These systems balance coordination and competition to optimize performance.
Example: In an e-commerce platform, some AI agents collaborate to recommend products based on user preferences (cooperative), while pricing bots from different sellers compete to offer the best price (competitive).
Hierarchical Multi Agent Systems
In hierarchical multi-agent systems, agents are structured at different levels, where higher-level agents oversee or coordinate lower-level agents. This setup improves decision-making efficiency by dividing tasks across different authority levels.
Example: In an autonomous warehouse, a central management system assigns tasks to different robotic workers. High-level agents handle global logistics, while lower-level agents focus on specific tasks like picking and sorting items.
Advantages of Multi Agent Systems
Multi agent systems offer a powerful approach to solving complex problems by distributing intelligence across multiple agents.
Scalability
Multi-agent systems can expand seamlessly by adding new agents without affecting overall performance. The agents function independently, allowing the system to handle increasing workloads, process large datasets, and manage complex environments efficiently.
Flexibility
MAS respond dynamically to changes in their environment without requiring manual intervention. Agents continuously adjust their behavior based on new data, evolving conditions, or unexpected disruptions. If an agent fails or a task changes, other agents can take over, ensuring smooth and uninterrupted operations.
Modularity
MAS are designed with a modular structure, meaning different tasks can be assigned to specific agents that operate independently while still being part of a unified system. This modularity simplifies maintenance, upgrades, and customization, allowing new capabilities to be integrated without affecting the entire system.
Specialization
Each agent in a MAS can be designed for a specific function, allowing for greater efficiency and precision. Specialized agents focus on their assigned tasks, leading to optimized performance, higher accuracy, and better resource allocation. In industries like healthcare, manufacturing, and cybersecurity, specialization helps improve decision-making and operational efficiency.
Collaborative Learning
MAS improve over time by learning from interactions with their environment and other agents. They share knowledge, refine strategies, and enhance problem-solving through experience. This ability to learn collectively is particularly useful in AI-driven systems that require continuous adaptation, such as financial trading algorithms or autonomous vehicles.
Parallel Processing
Since multiple agents work simultaneously on different tasks, multi-agent systems optimize computational and operational efficiency. Tasks can be executed in parallel rather than sequentially, allowing complex problems to be solved faster.
Robustness
MAS are designed to be resilient to failures. If one agent encounters an issue, others can compensate by taking over its responsibilities and preventing system-wide breakdowns.
Applications of Multi Agent Systems
Multi agent systems are transforming industries by handling complex, distributed, and dynamic environments. Here’s where they make an impact:
- Autonomous Vehicles & Robotics: These systems enable self-driving cars, drones, and robots to coordinate, adapt, and optimize navigation, collision avoidance, and task execution in real-time.
- Smart Grids & Energy: Intelligent agents balance energy loads, integrate renewables, and detect faults, enhancing power distribution and grid reliability.
- Healthcare & Medical Systems: Multi-agent systems improve hospital management, automate patient monitoring, and streamline drug discovery and telemedicine.
- Financial Markets & Trading: Trading bots analyze market trends, manage risks, and detect fraud, boosting efficiency and decision-making.
- Supply Chain & Logistics: Agents optimize inventory, transportation, and delivery by tracking shipments and adjusting routes dynamically.
- Smart Cities & Urban Planning: Intelligent agents manage traffic flow, waste collection, and public services, improving urban efficiency.
- Manufacturing & Industrial Automation: Multi-agent systems automate production lines, predict maintenance needs, and ensure smooth operations in smart factories.
Best Practices for Implementing Multi Agent Systems
Successfully building a multi agent system requires careful planning to ensure efficiency, adaptability, and reliability. Below are key best practices to follow:
Match the Architecture to the Problem
Choose an architecture that aligns with the system’s complexity and needs. Simple tasks may only require a single agent, while complex, dynamic environments benefit from a multi agent system. Based on the level of coordination and scalability required, decide between centralized, decentralized, or hybrid designs.
Start Simple and Improve Over Time
Develop the system with a basic structure and a limited number of agents. This allows for early testing, identifying potential issues, and refining the system before adding more complexity. Expanding gradually ensures better stability and control as the system evolves.
Clearly Define Agent Roles
Assign specific tasks to each agent to ensure efficiency and prevent conflicts. Specialization allows agents to focus on distinct responsibilities, improving performance and coordination. A well-organized system reduces redundancy and enhances collaboration.
Ensure Flexible Communication
Establish an effective communication method between agents to enable smooth coordination. Use structured communication for predictable workflows and dynamic communication for real-time adaptability. A well-designed system allows agents to exchange information efficiently while maintaining stability.
Balance Autonomy and Control
Strike a balance between giving agents independence and maintaining oversight to ensure coordination. Too much autonomy can lead to unpredictable behavior, while excessive control can reduce flexibility. Adjust the level of autonomy based on the system’s needs, allowing agents to make decisions when necessary while still adhering to overall system goals.
Design for Human-Agent Interaction
Create user-friendly interfaces that enable smooth interactions between agents and human users. Transparency in decision-making and clear feedback mechanisms improve usability and trust. A well-designed system should allow human intervention when needed.
Continuously Monitor Performance
Regularly evaluate system behavior to detect inefficiencies and refine functionalities. Implement tracking tools to analyze agent interactions and optimize their coordination over time. Continuous iteration keeps the multi-agent system responsive to evolving challenges.
Strengthen Security and Stability
Mitigate risks by implementing robust security measures, such as encryption and anomaly detection. Establish fail-safe mechanisms to ensure system continuity even when individual agents fail. A proactive approach enhances reliability and protects against vulnerabilities.
Final Thoughts
The rise of multi agent systems is redefining the way intelligent entities interact, adapt, and make decisions. From self-driving cars to financial modeling, these systems are powering the next wave of AI-driven automation.
With Astera’s visual drag-and-drop platform, businesses can easily design and deploy multi-agent systems without the need for complex coding. Astera simplifies AI-driven automation by enabling seamless integration, real-time data sharing, and intelligent decision-making—all within a unified environment.
The next frontier for multi agent systems lies in their integration with cutting-edge technologies—deep learning will enhance agent decision-making, blockchain will enable trust and security, and edge computing will bring real-time intelligence to the devices around us. As research pushes the boundaries of multi-agent systems, we are inching closer to a world where AI is not just reactive but proactively shaping our environments.
Are you ready to embrace the future of intelligent multi agent collaboration? Explore our product page to see how it works in action.