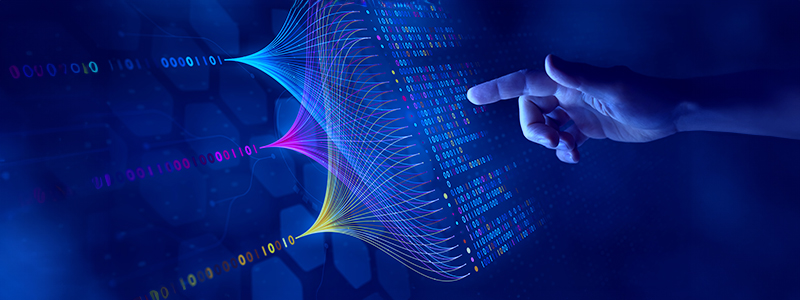
6 Crucial Questions to Help You Prepare Your Data For Analysis
Alexander Graham Bell’s motto, ‘Preparation is the key to success,’ resonates powerfully in data analysis. Raw data is an intricate puzzle—complex, unstructured, and scattered. Data preparation helps unravel the intricacies, transforming chaos into clarity. It involves refining, structuring, and formatting raw data, setting the stage for insightful analysis.
By preparing data for analysis, organizations can eliminate errors, ensure completeness and accuracy, and reveal hidden patterns, driving accurate analysis and informed decision-making. The significance of this preparatory phase cannot be emphasized enough; it’s the crucial step that paves the way for actionable intelligence.
Questions to Ask When Preparing Data for Analysis
As organizations delve into data preparation, they should consider the following questions to ensure their data is primed for analysis.
What is the main issue the analysis aims to solve?
Organizations must define the underlying business problem the analysis intends to address. This ensures that data preparation is laser-focused, aligning every subsequent step with the ultimate goal. Whether it’s optimizing supply chains, predicting customer behavior, or enhancing marketing strategies, a well-defined goal serves as a compass, guiding the course of data preparation endeavors.
Is the data accurate, complete, and suitable for the project?
Data quality is the foundation of reliable analysis. Anomalies and inconsistencies can lead to incorrect conclusions. Rigorous data cleansing and validation procedures are required to ensure consistency. The dataset should also be evaluated for accuracy, completeness, and relevance.
How are missing values and outliers being managed?
Addressing missing values and outliers is crucial for analysis integrity. Analysts should decide whether to omit, impute, or transform missing values and devise strategies for managing outliers that could potentially impact results. Proficiently handling these anomalies ensures dependable and accurate insights, a cornerstone for meaningful analysis.
Will any variables require transformation?
Data seldom presents itself in the ideal format for analysis. Variables may need to be transformed, normalized, or standardized to ensure meaningful comparisons and interpretations. The specific analytical goals and the underlying assumptions of the chosen analysis method should guide decisions regarding which variables to transform and how to do so.
Which documentation procedures ensure transparency?
Transparent documentation acts as a breadcrumb trail, guiding collaborators through the analytical process. Each step, from data cleaning to transformation, should be documented systematically. This documentation fosters accountability and enables others to follow and validate the approach, ensuring a transparent and replicable analysis.
How will the data preparation process be validated?
Validation serves as the litmus test for the data preparation process. Analysts must establish validation strategies to ascertain that the data is accurately transformed and manipulated. This often involves cross-validation techniques, comparing outcomes against established benchmarks, or conducting sensitivity analyses to assess the impact of various data preparation decisions.
The Importance of Preparing Data for Analysis
Embarking on data analysis without proper preparation sets up organizations for unreliable, misleading results. Here’s why preparing data for analysis is crucial:
Reliable Insights Depend on Clean Data
Well-prepared data is crucial for business intelligence. The conclusions drawn will be inaccurate if the data is messy or inaccurate, and vice versa. Data preparation ensures the data is accurate, consistent, and trustworthy, forming a solid basis for insightful findings.
It includes data cleansing, validation, and imputation, which collectively enhance the reliability of the conclusions drawn from the information.
Noise Reduction and Outlier Handling
Real-world data often contains irregularities like noise and outliers that can distort analysis results. Analysts can prevent outliers from skewing the overall conclusions by addressing them carefully. This process helps to focus the analysis on meaningful trends and patterns.
Noise reduction techniques, such as smoothing and filtering, help distinguish genuine patterns from random fluctuations. Outlier handling means identifying extreme data points that can unduly influence statistical measures and making informed decisions about their treatment.
Drawing Meaningful Comparisons
Variations in units, scales, or formats can hinder fair comparisons when dealing with data from different sources or timeframes. Data preparation standardizes these aspects, making it possible to draw accurate conclusions by ensuring consistency across the dataset.
Standardization ensures that data from diverse sources are transformed into a consistent format, facilitating meaningful comparisons. This step often includes converting units, harmonizing naming conventions, and aligning scales to create a unified dataset.
Time Savings
A significant portion of the analysis process is wrangling and cleaning data. Investing effort in data preparation upfront reduces troubleshooting during analysis, making the entire process more efficient.
Effective data preparation also saves valuable time in later stages of analysis by minimizing the need for repeated data fixes. It empowers analysts to focus on exploring insights and crafting meaningful visualizations, thereby enhancing the overall efficiency of the analytical workflow.
Guarding Against Bias
Preparing data for analysis presents an opportunity to detect and correct biases in the data. By addressing these biases proactively, enterprises can minimize their impact on the analysis results, ensuring a more objective and accurate interpretation.
Data scientists detect bias by examining the data for potential sources of bias, such as underrepresented groups or skewed samples. Data cleaning and transformation techniques can be applied to mitigate these biases, ensuring that the analysis provides a fair and unbiased representation of the underlying phenomena.
Boosting Model Performance
For predictive modeling to succeed, well-prepared data is essential. Techniques like normalization and feature engineering, part of data preprocessing, enhance the accuracy and robustness of models, leading to more reliable predictions.
Data preprocessing transforms raw data into a suitable format for machine learning algorithms. Normalization scales data to a consistent range, and feature engineering involves creating new relevant features from existing ones. Both steps contribute to improved model performance and more accurate predictions.
Point-and-click Data Prep With Astera
Organizations are increasingly recognizing the importance of well-prepared data in decision-making- be it strategic or tactical, to gain a competitive advantage. It is crucial for analysts to carefully assess and evaluate the information available and take the necessary steps to prepare the data for analysis. Accurate, complete data paves the way for actionable insights, strategic outcomes, and informed choices, consequently enhancing the operational efficiency, profitability, and, ultimately, the bottom line of enterprises.
Ready to elevate your data game? Dive into the future with a 14-day free trial of Astera and unlock the potential of seamless data preparation for your organization. Prepare your data in seconds with Astera’s intuitive interface and robust data profiling and cleansing features.