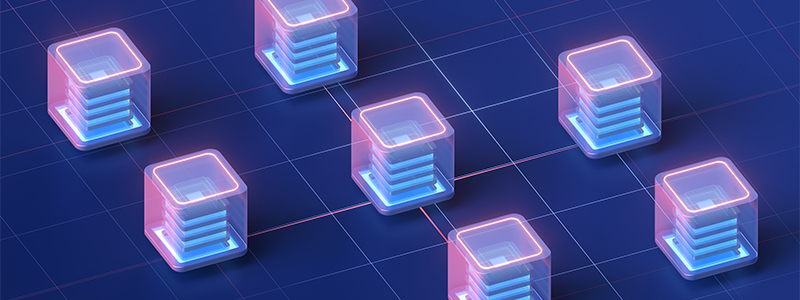
RAG: An X-Ray for Your Data
Retrieval Augmented Generation (RAG) is an intelligent assistant that helps you find exactly what you’re looking for in a pile of medical records. Like an X-ray shows you hidden details inside the body, RAG helps you quickly extract precise information from complex data.
RAG provides instant, accurate answers—often visualized in charts or summaries that require analysts to produce manually.
RAG combines two AI capabilities—retrieval systems and generative models. This means it pulls up documents, understands the context, and enhances the information, giving you relevant answers in seconds. Studies have shown that AI systems using retrieval-based models can reduce document search times by up to 70% compared to manual searches.
RAG’s Impact on Medicine
In an oncology study, doctors used an AI-powered system to sift through thousands of patient records to find treatment patterns for specific types of cancer. The retrieval system quickly pulled the relevant patient histories and treatment data. Then, the generative model summarized the findings, highlighting essential patterns such as the most effective treatments for patients with similar diagnoses. This process led to more precise recommendations and reduced manual search times by over 80%, allowing healthcare teams to spend more time on patient care rather than documentation.
A billing manager can also use RAG to ask, “How many claims were rejected last month?” or a hospital director can ask, “Which departments are seeing the highest patient throughput?” RAG’s generative model finds the data and delivers insights, complete with data visualizations like pie charts or bar graphs.
RAG Makes Data Extraction Faster & Smarter
RAG offers healthcare professionals a smarter and faster way to extract data from medical records.
-
Flexibility
RAG can handle various documents and formats and extract relevant information from unstructured documents without rigid templates. This versatility is like having a multi-tool that fits every data extraction scenario, helping you find the specific answers you need.
For healthcare administrators, this means handling everything from financial records and billing data to workforce management documents. For example, a hospital CFO could ask RAG, “What were the operational costs by the department for the last fiscal year?” and get a detailed department-by-department breakdown—no spreadsheets or manual data aggregation required.
-
Speed
RAG instantly searches and synthesizes information across different patient records. For instance, if you need to quickly understand a patient’s medical history before surgery or treatment, RAG pulls the relevant information. It delivers a concise, accurate overview—saving you hours of manual searching. It speeds up the diagnostic process, giving you more time to focus on treatment.
-
Accuracy
RAG uses advanced AI models to filter out irrelevant information and pinpoint exactly what you want. This precision ensures you rely on accurate, contextually relevant data, whether diagnosing a condition or preparing a treatment plan.
-
Lower Cost
RAG reduces the need for human intervention, allowing healthcare organizations to lower operational costs while maintaining high efficiency and accuracy.
How to Use RAG to Extract Data from Medical Records (Spoiler Alert: It’s Not Brain Surgery)
RAG follows three basic steps to process and extract information from medical records.
Step One: Retrieval
The system first indexes the available data, creating a “map” of all the documents—medical records, lab results, prescriptions, etc. When a healthcare professional inputs a query (for example, “What’s the best treatment for a patient with X condition?”), the RAG framework dives into this mapped data and pulls out the most relevant pieces.
Step Two: Augmentation
RAG uses search and ranking algorithms to rank the retrieved documents based on relevance.
Search algorithms are used to hunt for information across a large dataset. They work like Google, where you type in a question, and the system finds all the documents related to that question. Then, ranking algorithms prioritize the documents by relevance so the most useful, on-topic documents appear first.
Step Three: Generation
Finally, after gathering and enhancing the information, RAG delivers actionable insights.
RAG processes all retrieved documents to create a clear, concise answer to the query. Its generative model summarizes the extracted data in plain language.
For example, instead of simply listing five studies related to a condition, RAG could provide a summary that combines key findings, compares treatment options, and highlights the most effective approach—all in response to a single query.
Conclusion
RAG is a smart and practical solution that cuts through the noise of complex medical records to get you the most relevant and accurate data. It simplifies decision-making by combining generative and retrieval systems with generative AI.
At Astera, we provide an easy-to-use, no-code interface that automates the entire process, from document indexing to retrieving context-driven answers. Our solution can help you explore patient histories, navigate complex research documents, and quickly access the necessary information.
Start a free trial today to see how Astera can streamline your workflow.
Ready to speed up your medical data retrieval process? Try our no-code solution and see how RAG can instantly extract and enhance insights from your medical records. Start your free trial now!Get Started with Faster Data Extraction Today